AI-Powered Podcast Creation: Analyzing Repetitive Scatological Documents For Engaging Content
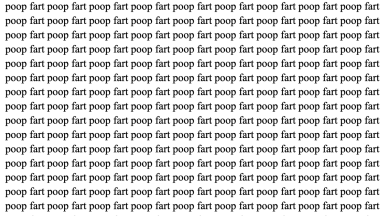
Table of Contents
Data Acquisition and Preparation for AI Analysis
Sourcing Repetitive Scatological Documents
The ethical considerations surrounding data acquisition are paramount. Sourcing repetitive scatological documents requires careful planning and adherence to strict privacy regulations. Potential sources, after appropriate anonymization, could include anonymized medical records used for research purposes, literary analysis of specific genres focusing on scatological themes, or even anonymized online forums dedicated to certain topics (with explicit consent). Data anonymization is crucial; techniques like differential privacy and k-anonymity can help protect individual identities while preserving the data's utility.
- Data Cleaning and Preprocessing: Before any AI analysis, rigorous data cleaning is essential. This involves removing irrelevant characters, handling missing values, and correcting inconsistencies in formatting.
- Data Standardization and Format Conversion: The data needs to be standardized into a consistent format suitable for AI processing. This might involve converting text to a structured format like JSON or converting different text encodings.
- Tools and Techniques: Regular expressions are invaluable for cleaning text data, identifying and removing unwanted patterns, and transforming the data into a usable format. Python libraries like
re
are commonly used for this purpose. This stage of AI data analysis is crucial for accurate results.
AI Algorithms for Pattern Recognition and Theme Extraction
Natural Language Processing (NLP) Techniques
Natural Language Processing (NLP) is the core technology enabling the analysis of these documents. NLP techniques unlock the hidden patterns and themes within the seemingly random data.
- Sentiment Analysis: This determines the overall emotional tone of the text, identifying prevalent feelings associated with specific topics or events.
- Topic Modeling (LDA): Latent Dirichlet Allocation (LDA) is a powerful technique for discovering underlying topics within a collection of documents, revealing hidden connections and themes.
- Named Entity Recognition (NER): NER identifies and classifies named entities like people, organizations, locations, and medical conditions, allowing for the identification of key players or concepts.
- AI Tools and Libraries: Libraries like spaCy and NLTK provide pre-trained models and tools for performing these NLP tasks efficiently. The power of machine learning for podcasting lies in the ability to automate these processes.
Transforming Data Insights into Podcast Episodes
Developing Podcast Concepts
Once the AI has extracted themes and patterns, the human element takes over. The extracted information provides a foundation for creative podcast episodes.
- Unexpected Insights: Repetitive scatological documents, while seemingly bizarre, might reveal unexpected insights into human behavior, societal norms, or historical trends. For example, patterns in language use might reflect cultural shifts or evolving attitudes towards certain subjects.
- Compelling Narratives: The challenge lies in transforming raw data into engaging narratives. This requires a creative approach to storytelling, weaving together the AI-generated insights into a cohesive and compelling podcast episode.
- Human Creativity: AI acts as a tool, providing the raw material. Human creativity is crucial in shaping this material into a well-structured and captivating podcast. AI-driven topic discovery is just the first step in the podcast content generation process.
Ethical Considerations and Responsible AI Use
Data Privacy and Anonymization
Ethical considerations are paramount throughout the entire process. Data privacy must be rigorously protected.
- Data Anonymization: Robust anonymization techniques are crucial to prevent the identification of individuals. This should be a top priority in podcast data mining.
- Bias Mitigation: AI models can inherit biases present in the data. It's crucial to identify and mitigate these biases to ensure fair and unbiased podcast content.
- Transparency: The AI-powered podcast creation process should be transparent, with clear explanations of the data sources and the AI techniques used. Responsible AI necessitates openness and accountability.
Conclusion
Using AI to analyze repetitive scatological documents for podcast content involves careful data acquisition, meticulous preprocessing, powerful NLP techniques, and creative storytelling. The process highlights the potential for discovering unique and engaging storylines from unconventional sources. AI-powered podcast ideas are limited only by the imagination of the creator. Start experimenting with AI-powered podcast creation today! Unlock the power of unconventional data sources and discover new avenues for engaging your audience. The future of podcasting is here, and it's powered by AI.
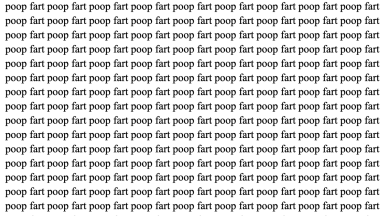
Featured Posts
-
Justin Herbert Leads Chargers To Brazil For 2025 Season Opener
Apr 27, 2025 -
Bencic Madre Campeona Un Regreso Triunfal A Las Pistas
Apr 27, 2025 -
Alberto Ardila Olivares Estrategia Y Garantia De Gol
Apr 27, 2025 -
2025 Nfl Season Chargers And Justin Herbert Head To Brazil
Apr 27, 2025 -
I Preordered My Switch 2 At Game Stop The Offline Experience
Apr 27, 2025
Latest Posts
-
Paolini Y Pegula Fin De Su Participacion En El Wta 1000 De Dubai
Apr 27, 2025 -
Eliminacion De Paolini Y Pegula En El Wta 1000 De Dubai
Apr 27, 2025 -
Dubai 2024 Paolini Y Pegula Fuera Del Wta 1000
Apr 27, 2025 -
Government Appoints Vaccine Skeptic To Lead Immunization And Autism Study
Apr 27, 2025 -
Wta 1000 Dubai Caida Inesperada De Paolini Y Pegula
Apr 27, 2025