AI's "Thinking" Process: Simple Algorithms, Complex Results
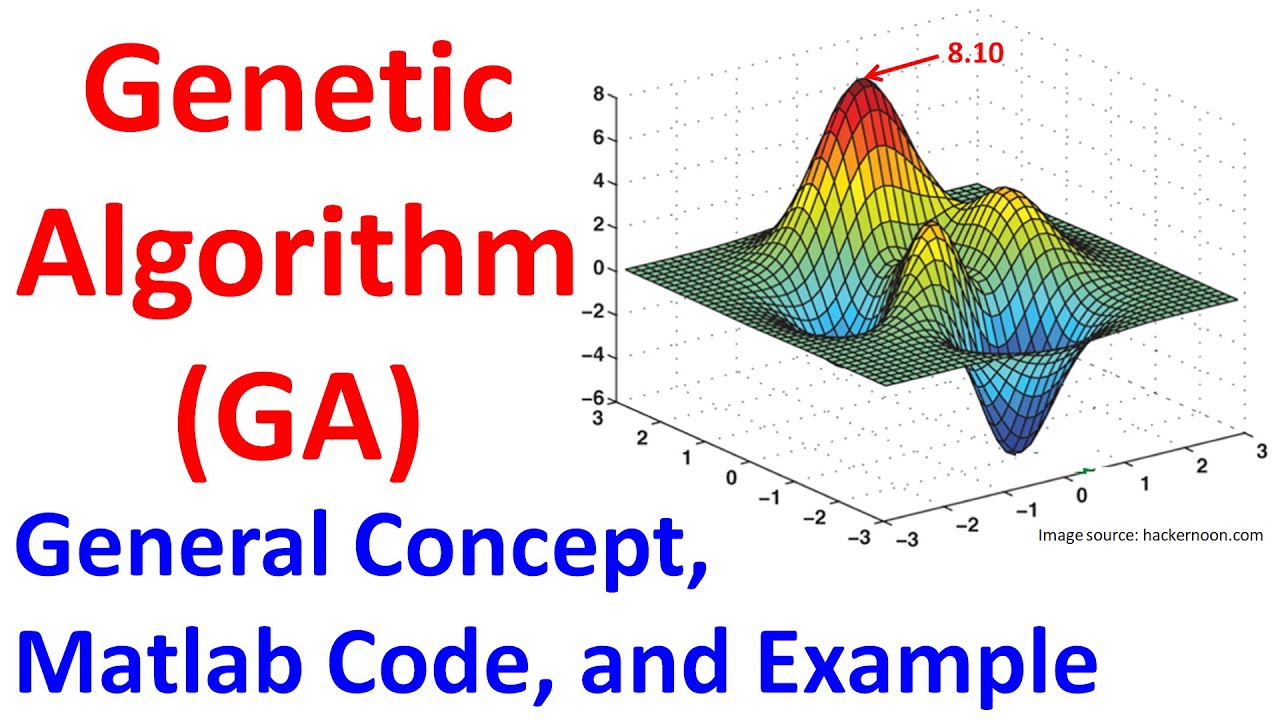
Table of Contents
The Building Blocks: Understanding Basic AI Algorithms
AI's "thinking" isn't driven by human-like consciousness, but rather by sophisticated algorithms processing vast amounts of data. Let's examine the fundamental building blocks:
Supervised Learning: Learning from Labeled Data
Supervised learning algorithms learn from labeled data, where each data point is tagged with the correct answer. Think of it like a teacher showing a student examples and providing the correct labels. This approach is widely used in various applications:
- Training data: The labeled dataset used to train the algorithm.
- Features: The specific characteristics of the data used for learning (e.g., pixels in an image, words in a sentence).
- Classification: The process of assigning data points to predefined categories (e.g., classifying images as "cat" or "dog").
- Accuracy: A measure of how well the algorithm correctly classifies new, unseen data.
Examples include image recognition (identifying objects in photos) and spam filtering (classifying emails as spam or not spam). Effective supervised learning algorithms, like Support Vector Machines (SVMs) and decision trees, rely on meticulously prepared AI training data
to achieve high accuracy in supervised learning algorithms
.
Unsupervised Learning: Discovering Hidden Patterns
Unlike supervised learning, unsupervised learning algorithms work with unlabeled data, seeking to identify inherent structures and patterns. This is like giving a child a box of toys and letting them discover how to categorize them on their own. Applications include:
- Clustering: Grouping similar data points together (e.g., segmenting customers based on purchasing behavior).
- Dimensionality reduction: Simplifying complex data by reducing the number of variables while preserving important information.
- Association rule mining: Discovering relationships between different variables (e.g., finding products frequently bought together in a supermarket).
Unsupervised AI
techniques, such as k-means clustering and principal component analysis, are powerful tools for pattern recognition algorithms
, allowing us to extract valuable insights from large, unstructured datasets.
Reinforcement Learning: Learning Through Trial and Error
Reinforcement learning algorithms learn through trial and error, interacting with an environment and receiving rewards or penalties based on their actions. This is similar to how humans learn through experience. Examples include:
- Rewards: Positive feedback for desirable actions.
- States: The different situations the AI can find itself in.
- Actions: The choices the AI can make.
- Policy: The strategy the AI learns to maximize rewards.
Reinforcement learning powers game-playing AI like AlphaGo and is crucial for training robots to perform complex tasks. These reinforcement learning algorithms
are at the forefront of AI decision-making
capabilities.
From Simple Rules to Complex Behavior: Emergence and Unexpected Results
The true magic of AI lies not just in individual algorithms, but in their intricate interactions.
The Power of Networks: Emergence from Simple Components
The interconnectedness of simple algorithms, especially in neural networks, gives rise to complex behavior. This is the concept of emergence:
- Neural networks: Systems of interconnected nodes (neurons) that process information in parallel.
- Deep learning: A type of neural network with multiple layers, capable of learning highly complex patterns.
- Emergent properties: Complex behaviors arising from the interaction of simpler components.
Neural network algorithms
and deep learning models
are revolutionizing various fields, demonstrating the power of AI emergence
to solve complex problems.
Handling Uncertainty and Noise: Robustness in the Face of Imperfect Data
Real-world data is rarely perfect; it's often incomplete or noisy. AI algorithms must be robust enough to handle this uncertainty:
- Probability: Using probabilistic models to represent uncertainty.
- Bayesian methods: A powerful framework for updating beliefs based on new evidence.
- Robustness: The ability of an algorithm to perform well despite noisy or incomplete data.
Effective AI uncertainty
management is essential, and techniques like Bayesian inference and robust statistical methods are crucial for developing robust algorithms
capable of handling noise handling
effectively.
Bias and Limitations: Ethical Considerations in AI Development
AI systems are not immune to bias; they can inherit biases present in the data they are trained on or in the algorithms themselves:
- AI bias: Systematic errors in AI systems that reflect and amplify existing societal biases.
- Ethical AI: The development and deployment of AI systems that are fair, transparent, and accountable.
- Responsible AI development: Prioritizing ethical considerations throughout the AI lifecycle.
Addressing AI bias
is crucial for the development of ethical AI
and responsible AI development
. Careful consideration of data sources and algorithmic design is essential to minimize bias and ensure fairness.
Applications and Examples of AI's "Thinking" Process in Action
AI's "thinking" process is transforming various sectors:
Real-world examples:
AI's capabilities span healthcare (disease diagnosis), finance (fraud detection), transportation (self-driving cars), and more.
Focus on specific applications:
- Healthcare: AI algorithms, particularly deep learning models, analyze medical images to detect diseases like cancer with high accuracy.
- Finance:
AI in finance
utilizes machine learning algorithms for fraud detection and risk assessment, improving efficiency and accuracy. - Transportation: Self-driving cars rely on a combination of computer vision, reinforcement learning, and other
AI algorithms
for navigation and decision-making. These systems must make complex decisions in real-time, demonstrating the sophistication ofAI's thinking process
.
Conclusion
AI's "thinking" process, though based on relatively simple algorithms, produces surprisingly complex and sophisticated results. The interaction of these algorithms, along with emergent properties and advanced techniques for handling uncertainty, leads to impressive capabilities across various fields. The contrast between the simple building blocks and the complex outcomes remains a compelling aspect of AI. Want to delve deeper into the fascinating world of AI algorithms and their unexpected capabilities? Explore [link to relevant resource]. Or share your thoughts on how simple algorithms can generate complex results in the comments below!
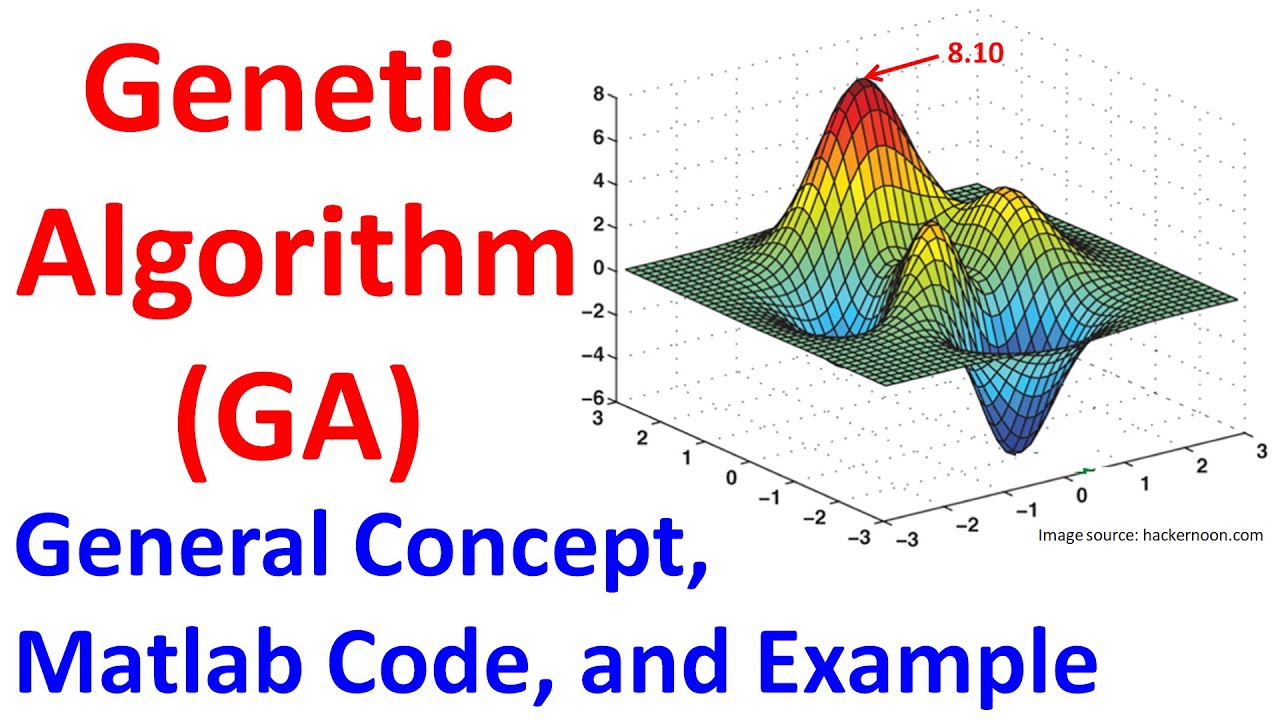
Featured Posts
-
Trumps Executive Order Nationwide List Of Sanctuary Cities And States
Apr 29, 2025 -
Are High Stock Prices A Worry Bof As Analysis Of Market Valuations
Apr 29, 2025 -
Cnn Alan Cumming Shares Beloved Childhood Memory From Scotland
Apr 29, 2025 -
Analyzing Trumps Next 100 Days Trade Deals Deregulation And Executive Orders
Apr 29, 2025 -
The Dysprosium Crisis Challenges And Opportunities In The Ev Revolution
Apr 29, 2025
Latest Posts
-
Reflecting On A Happy Day February 20 2025
Apr 29, 2025 -
Investigation Reveals Fatal Mistakes In Black Hawk And American Airlines Crash
Apr 29, 2025 -
Garantia De Gol Con Alberto Ardila Olivares
Apr 29, 2025 -
Alberto Ardila Olivares Garantia De Gol
Apr 29, 2025 -
How To Have A Happy Day On February 20 2025
Apr 29, 2025