Build Voice Assistants Easily With OpenAI's New Tools
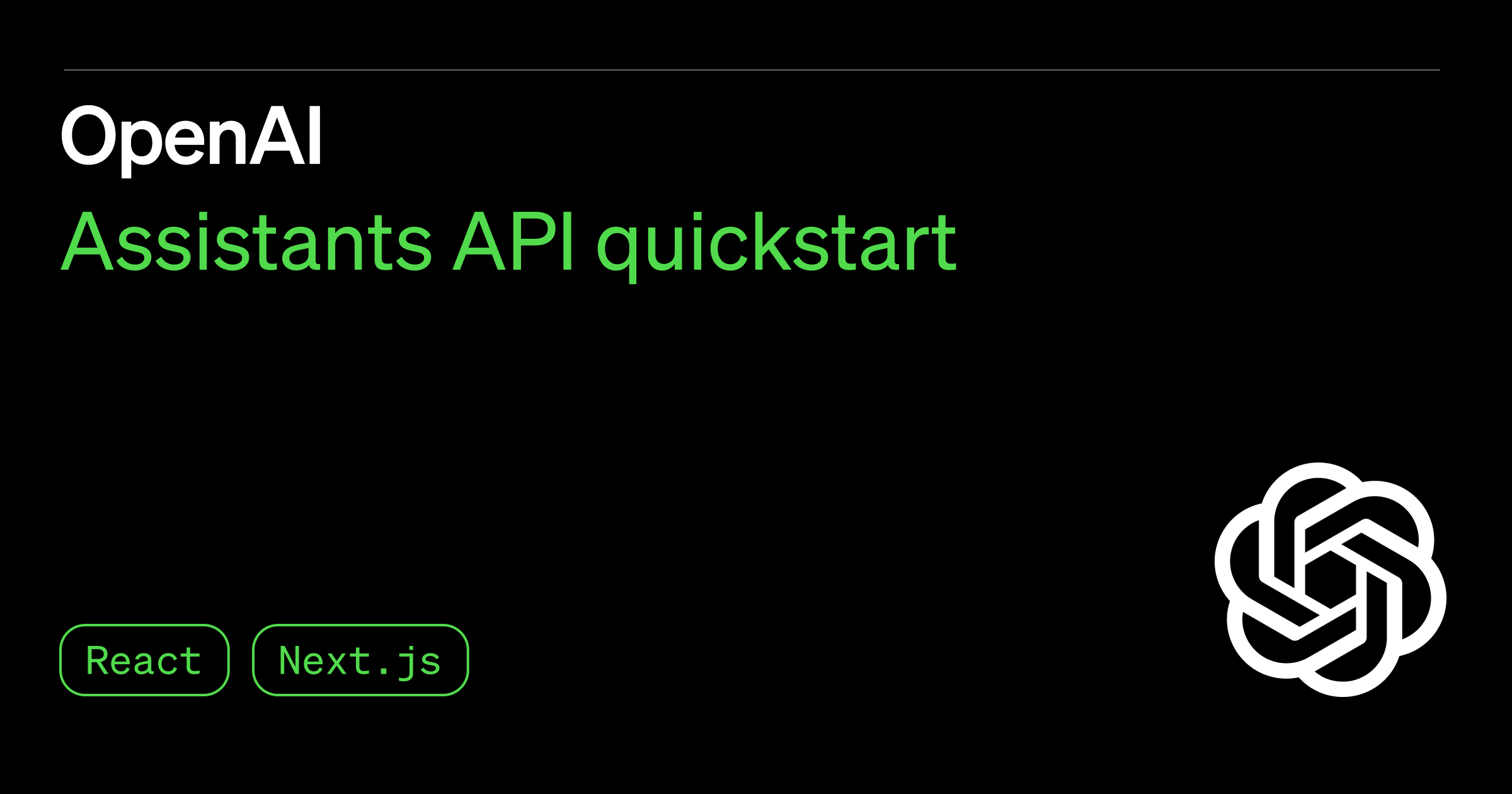
Table of Contents
Accessing and Understanding OpenAI's Relevant APIs
Building a voice assistant with OpenAI hinges on effectively utilizing several key APIs. These APIs handle different aspects of voice interaction, from converting speech to text to understanding and responding to user requests. Mastering these APIs is the cornerstone of successful voice assistant development.
Keywords: OpenAI API, API integration, speech-to-text API, text-to-speech API, OpenAI Whisper, OpenAI Codex
-
Key APIs: OpenAI offers several crucial APIs for building voice assistants:
- Whisper: This powerful speech-to-text API converts audio input into text, forming the foundation for understanding user commands. It's known for its accuracy and ability to handle various accents and audio qualities.
- OpenAI Codex/GPT Models: These large language models (LLMs) are central to natural language understanding (NLU). They interpret the transcribed text, understand the user's intent, and generate appropriate responses.
- Text-to-Speech APIs (Third-Party): While OpenAI doesn't directly offer a text-to-speech API, several robust third-party options integrate seamlessly with their models, allowing your voice assistant to speak back to the user. Examples include Amazon Polly, Google Cloud Text-to-Speech, and Microsoft Azure Text-to-Speech. You'll need to obtain API keys from these providers separately.
-
API Key Generation and Authentication: Access to OpenAI's APIs requires obtaining an API key from your OpenAI account. This key is crucial for authenticating your requests and managing your usage. Detailed instructions for generating and managing your API keys are available in the OpenAI documentation.
-
Ease of Integration: OpenAI's APIs are designed for easy integration with various programming languages. Python is a particularly popular choice due to its extensive libraries and strong support for AI/ML tasks. We'll focus on Python examples in this guide.
Handling Speech-to-Text Conversion with Whisper
Whisper's accurate and efficient speech recognition is vital to your voice assistant's functionality. Understanding its capabilities and limitations is crucial for building a robust system.
Keywords: Whisper API, speech recognition, real-time transcription, audio processing
-
Whisper's Capabilities: Whisper excels at transcribing speech across various accents and audio conditions. It supports multiple languages and handles noisy audio surprisingly well.
-
Transcribing User Voice Input: The process generally involves sending the audio file (various formats supported, including WAV, MP3, etc.) to the Whisper API. The API returns a transcribed text string.
-
Handling Different Audio Formats and Noise Reduction: Whisper can handle various audio formats. Preprocessing, such as noise reduction, can improve accuracy. Libraries like Librosa in Python are helpful for audio manipulation before sending it to Whisper.
Leveraging OpenAI's Language Models for Natural Language Understanding
The heart of your voice assistant lies in its ability to understand and respond to user requests. OpenAI's GPT models excel at this task.
Keywords: GPT models, natural language understanding (NLU), intent recognition, entity extraction, dialogue management
-
Intent Recognition and Entity Extraction: GPT models analyze the transcribed text to determine the user's intent (what they want to do) and extract relevant entities (specific pieces of information). For example, in the request "Set a timer for 15 minutes," the intent is "set timer," and the entity is "15 minutes."
-
Building Conversational Flows and Dialogue Management: Designing effective dialogue management is essential for creating a natural and engaging experience. This involves structuring conversations, handling unexpected inputs, and maintaining context across multiple turns.
-
Code Snippets (Python Example): A basic Python interaction with a GPT model might look like this (replace with your actual API key and prompt):
import openai
openai.api_key = "YOUR_API_KEY"
response = openai.Completion.create(
engine="text-davinci-003", # or other suitable model
prompt="What is the capital of France?",
max_tokens=150,
n=1,
stop=None,
temperature=0.7,
)
print(response.choices[0].text.strip())
Designing Engaging Conversational Experiences
Creating a truly engaging voice assistant requires careful consideration of the user experience (UX). A well-designed conversational flow is as important as the underlying technology.
Keywords: conversational design, user experience (UX), voice user interface (VUI), dialogue flow, prompt engineering
-
Intuitive Conversational Flows: Design your conversations to be straightforward and easy to follow. Avoid complex menus or confusing language. Keep interactions concise and focused.
-
Effective Prompts: The way you prompt the user for information significantly impacts the quality of their input. Clearly defined prompts minimize misunderstandings and improve overall interaction.
-
Handling Unexpected Inputs: Users may not always provide perfectly formatted requests. Robust error handling and fallback mechanisms are essential.
-
Maintaining a Natural Conversational Tone: Strive for a conversational tone that feels natural and human-like, rather than robotic.
Integrating Text-to-Speech for Natural Output
Once your voice assistant understands the user's request and generates a response, you need to convert the text into audible speech.
Keywords: text-to-speech (TTS), natural-sounding voice, voice synthesis, speech synthesis API
-
Integrating Text-to-Speech APIs: Numerous third-party TTS APIs offer high-quality voice synthesis. Choose one that best suits your needs in terms of voice quality, language support, and cost.
-
Voice Selection and Speech Parameter Control: Most TTS APIs provide options to select different voices, adjust speech speed, pitch, and intonation for a more personalized and engaging experience.
-
Code Examples (Python Example - Illustrative): Integrating a TTS API (you'll need to replace placeholders with your API key and specifics of the chosen API):
# Illustrative, replace with your chosen TTS API's library and methods
from some_tts_library import TextToSpeech
tts = TextToSpeech(api_key="YOUR_TTS_API_KEY")
audio_data = tts.synthesize_speech("Hello, this is your voice assistant.")
# ...save or stream audio_data...
Deploying and Scaling Your Voice Assistant
Finally, you need to deploy your voice assistant and prepare it to handle a potentially large number of users.
Keywords: deployment, cloud platforms, scalability, serverless functions, voice assistant deployment
-
Deployment Options: Cloud platforms like AWS, Google Cloud, and Azure provide robust infrastructure for deploying and scaling voice assistants.
-
Scalability: Design your architecture to handle increased user traffic without performance degradation. Consider using serverless functions or containerization technologies like Docker for efficient resource management.
-
Serverless Functions: Serverless functions are particularly well-suited for voice assistants, allowing you to scale automatically based on demand. This avoids the need to manage servers directly.
Conclusion
OpenAI's powerful and accessible tools have dramatically simplified the process of building voice assistants. By leveraging the capabilities of the OpenAI API, including Whisper for speech-to-text and GPT models for natural language understanding, developers can create sophisticated and engaging voice assistants with significantly reduced effort. This guide has provided a foundational understanding of how to utilize these tools effectively, from designing intuitive conversational flows to deploying and scaling your application. Start building your own voice assistant today using OpenAI's innovative technology! Explore the OpenAI API and unlock the potential of building your own cutting-edge voice assistant.
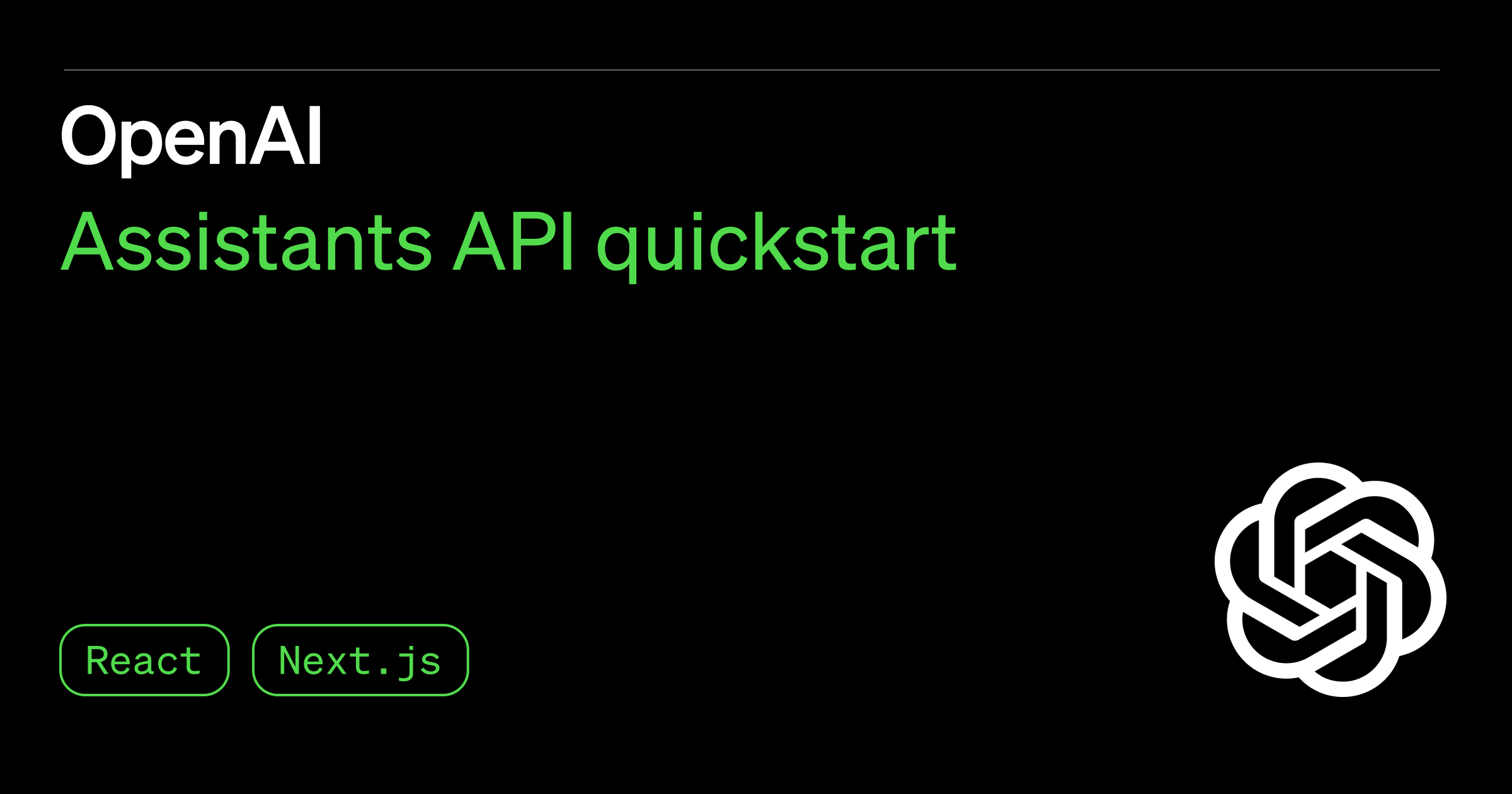
Featured Posts
-
Will Minnesota Film Tax Credits Attract More Productions
Apr 29, 2025 -
Tabilo Upsets Djokovic In Straight Sets At Monte Carlo Masters 2025
Apr 29, 2025 -
The Rise Of Huawei A New Contender In The Ai Chip Market With Its Exclusive Design
Apr 29, 2025 -
Data Breach Exposes Millions In Losses Office365 Security Failure Investigated
Apr 29, 2025 -
Will Tax Incentives Spur Film And Television Growth In Minnesota
Apr 29, 2025
Latest Posts
-
Unreported Aspects Of The January 29th Dc Air Disaster A Critique Of The Ny Times Coverage
Apr 29, 2025 -
Donald Trump To Pardon Pete Rose Mlb Hall Of Fame Induction Call
Apr 29, 2025 -
The January 29th Dc Air Disaster A Comparison Of News Coverage Including The Ny Times
Apr 29, 2025 -
Analyzing The Ny Times Handling Of The January 29th Dc Air Disaster Story
Apr 29, 2025 -
Trump To Pardon Pete Rose Posthumously Examining The Implications
Apr 29, 2025