CNIL's AI Guidelines: Practical Steps For Businesses In The EU
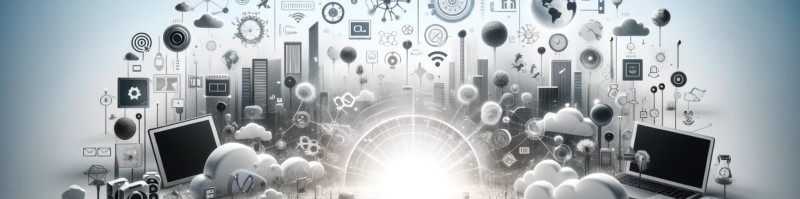
Table of Contents
Understanding the Scope of CNIL's AI Guidelines
The CNIL AI Guidelines aren't a standalone regulation but rather a practical interpretation of existing laws, primarily the GDPR, in the context of AI. They apply broadly to various AI systems and business types operating within the EU. Let's clarify their scope:
- Definition of AI systems covered: The guidelines encompass a wide range of AI technologies, including machine learning (ML), deep learning (DL), natural language processing (NLP), computer vision, and robotic process automation (RPA). Essentially, any system using algorithms to make decisions or predictions falls under consideration.
- Types of businesses impacted: The guidelines impact all businesses using AI systems, regardless of size – from small and medium-sized enterprises (SMEs) to large corporations. The complexity of implementation might differ based on the size and sophistication of the AI system, but the underlying principles remain consistent.
- Specific sectors addressed: While not sector-specific, the implications of the guidelines are felt across all industries. However, sectors like healthcare, finance, and law enforcement, where AI decisions have significant consequences, face enhanced scrutiny. The use of AI in recruitment, customer service, and risk assessment all fall under the scope.
- Relationship with GDPR and other EU regulations: The CNIL AI Guidelines are deeply intertwined with the GDPR. They clarify how the principles of data protection, such as purpose limitation, data minimization, and accountability, apply to AI systems. They also consider the implications of other relevant EU regulations, such as the AI Act (once finalized).
Data Protection by Design and Default in AI Systems
A core tenet of the CNIL AI Guidelines is the principle of data protection by design and by default. This means that data protection must be integrated into the design and development of AI systems from the outset, and the system should only process the minimum amount of data necessary.
- Minimizing data collection for AI training: Only collect data absolutely necessary for the specific AI application. Avoid unnecessary data collection or over-collection.
- Data anonymization and pseudonymization techniques: Implement robust anonymization and pseudonymization strategies to protect personal data. This might involve techniques like differential privacy or data masking.
- Ensuring data security throughout the AI lifecycle: Implement strong security measures to protect data throughout the entire lifecycle of the AI system, from collection to disposal. This includes data encryption, access controls, and regular security audits.
- Implementing appropriate technical and organizational measures: This involves establishing appropriate technical and organizational measures to ensure data protection and compliance with GDPR, including data breach notification protocols.
- Examples of best practices for data minimization in specific AI applications: For example, in facial recognition, using only necessary facial features instead of the entire image. In natural language processing, using only relevant portions of text instead of full documents.
Transparency and Explainability in AI
The CNIL AI Guidelines strongly emphasize the need for transparency and explainability in AI systems. Users should understand how AI systems process their data and the logic behind decisions that affect them.
- Providing users with clear information about how AI systems process their data: Provide concise, accessible information about the data used, the purpose of processing, and the user's rights.
- Documenting the AI system's decision-making process: Maintain detailed records of the AI system's logic, algorithms, and data sources to allow for auditing and explainability.
- Implementing mechanisms for users to challenge AI-driven decisions: Establish clear procedures for users to contest AI-driven decisions, including the right to human intervention.
- Addressing the "black box" problem in AI: Work towards developing AI systems that are not "black boxes," promoting interpretable and explainable AI (XAI) techniques.
- Techniques for improving the transparency of AI algorithms: This could involve using simpler algorithms, providing visualizations of the decision-making process, or employing model explainability techniques like LIME or SHAP.
Human Oversight and Control in AI Systems
Maintaining human oversight and control over AI systems is crucial, to ensure ethical and responsible AI deployment and prevent unintended biases or consequences.
- Defining roles and responsibilities for human oversight: Clearly define who is responsible for overseeing the AI system, its development, deployment, and ongoing monitoring.
- Establishing mechanisms for human intervention in AI decision-making: Implement mechanisms that allow humans to intervene in or override AI decisions, particularly in high-stakes situations.
- Regularly auditing AI systems for bias and fairness: Conduct regular audits to identify and mitigate potential biases in the AI system's data, algorithms, and outcomes.
- Implementing procedures for handling complaints and disputes: Establish clear procedures for handling complaints related to AI-driven decisions.
- The importance of human-in-the-loop systems: Prioritize the use of human-in-the-loop systems where human intervention and oversight are integral to the AI system's operation.
Practical Steps for Compliance with CNIL's AI Guidelines
Implementing the CNIL AI Guidelines requires a proactive and comprehensive approach. Here are some practical steps businesses can take:
- Conducting a data protection impact assessment (DPIA): Perform a DPIA to identify and assess potential risks to individuals' rights and freedoms associated with the use of the AI system.
- Developing an AI ethics policy: Create a clear AI ethics policy that outlines the principles and guidelines for responsible AI development and deployment.
- Training employees on AI ethics and data protection: Provide training to employees on the ethical considerations and legal requirements related to AI and data protection.
- Implementing robust data governance procedures: Establish strong data governance procedures to ensure data quality, security, and compliance.
- Regularly reviewing and updating AI systems to maintain compliance: Regularly review and update your AI systems to ensure they continue to comply with evolving regulations and best practices.
Conclusion
Successfully navigating the complex landscape of AI regulation in the EU requires a thorough understanding of the CNIL AI Guidelines. By implementing the practical steps outlined in this article, businesses can ensure compliance with data protection laws, mitigate risks, and build trust with their customers. Staying informed about updates to the CNIL AI Guidelines and proactively adapting your AI systems is crucial for long-term success. Take the first step towards CNIL AI compliance today!
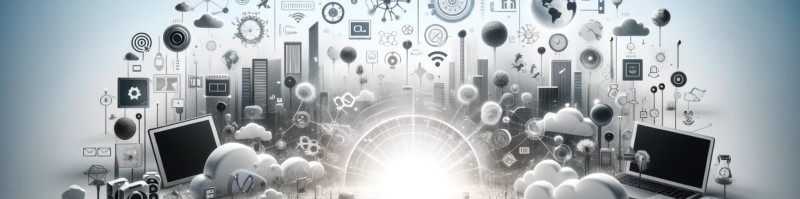
Featured Posts
-
Planification Des Places Pour Les Funerailles Du Pape Complexites Et Considerations
Apr 30, 2025 -
Schneider Electric Invests In Education New Center Of Excellence At Vignan University
Apr 30, 2025 -
Analysis Trumps Speech To Congress And Its Impact
Apr 30, 2025 -
Ru Pauls Drag Race Live 1000th Show Global Livestream Event
Apr 30, 2025 -
Significant Changes In Dance Marchs Roster Overview
Apr 30, 2025
Latest Posts
-
Analysis Trumps Speech To Congress And Its Impact
Apr 30, 2025 -
Trump Weighs In On Us Canada Ties Ahead Of Canadian Election
Apr 30, 2025 -
Trumps First Congressional Speech Key Issues And Expectations
Apr 30, 2025 -
Us Canada Relations Trumps Remarks In The Days Before The Canadian Election
Apr 30, 2025 -
Trumps Address To Congress A Divided Nation Awaits
Apr 30, 2025