Current AI Thinking: Capabilities And Constraints
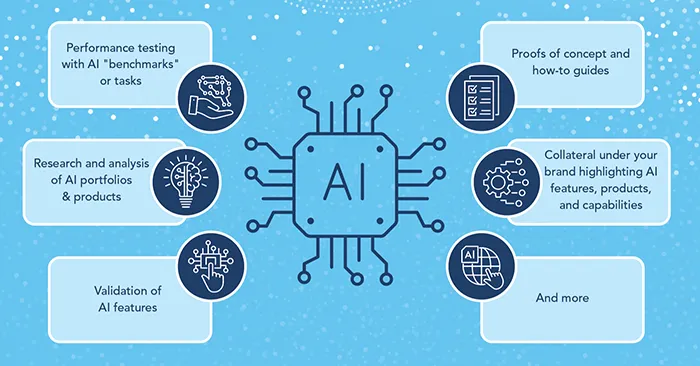
Table of Contents
The Impressive Capabilities of Current AI
Current AI thinking is largely focused on harnessing the power of machine learning and deep learning to solve complex problems. These advancements have led to remarkable progress across numerous sectors.
Machine Learning and Deep Learning Breakthroughs
Machine learning (ML) involves algorithms that allow computers to learn from data without explicit programming. Deep learning (DL), a subset of ML, utilizes artificial neural networks with multiple layers to analyze data and extract complex patterns. These breakthroughs have fueled significant progress in:
- Image Recognition: AI systems now surpass human accuracy in identifying objects, faces, and patterns in images, with applications in medical diagnosis, security, and autonomous driving.
- Natural Language Processing (NLP): AI can understand, interpret, and generate human language, powering chatbots, machine translation tools, and sentiment analysis applications.
- Predictive Analytics: AI algorithms can analyze historical data to predict future trends, enabling better decision-making in finance, marketing, and healthcare.
Specific achievements include:
- Medical Diagnosis: AI aids in early cancer detection, improving diagnostic accuracy and efficiency.
- Financial Modeling: AI optimizes investment strategies and fraud detection systems.
- Autonomous Vehicles: Self-driving cars rely heavily on AI for navigation, object recognition, and decision-making.
Limitations: Despite these successes, current ML and DL models suffer from limitations like data bias, leading to inaccurate or unfair outcomes, and a lack of generalizability, meaning they often struggle to adapt to new, unseen data.
AI's Enhanced Problem-Solving Abilities
Current AI thinking also highlights AI's role in optimizing complex processes and automating tasks. AI is increasingly used to:
- Optimize Supply Chains: Predictive models improve inventory management, logistics, and delivery efficiency.
- Detect Fraud: AI algorithms identify suspicious transactions and patterns to prevent financial crimes.
- Personalize Medicine: AI helps tailor treatments based on individual patient characteristics and genetic information.
These applications significantly improve efficiency and productivity. However, the automation potential of AI raises concerns about job displacement and the need for workforce adaptation and retraining programs. Current AI thinking must address these societal implications proactively.
Significant Constraints Facing Current AI Systems
While the capabilities of AI are impressive, significant constraints remain. Current AI thinking must acknowledge and address these limitations for responsible development and deployment.
Data Dependency and Bias
AI systems heavily rely on data for training. The quality and representativeness of this data are crucial. Biased datasets, reflecting societal prejudices, can lead to AI systems perpetuating and even amplifying these biases. Efforts to mitigate bias include:
- Data Augmentation: Expanding datasets to include more diverse and representative samples.
- Algorithmic Fairness: Developing algorithms that are less susceptible to bias and promote equitable outcomes.
Lack of Generalizability and Explainability
Current AI systems often struggle to generalize their learning to new, unseen situations or tasks. Many AI algorithms, particularly deep learning models, are "black boxes," making it difficult to understand their decision-making processes. This lack of explainability hinders trust and accountability. Research in explainable AI (XAI) aims to make AI models more transparent and understandable.
Ethical and Societal Concerns
The rapid advancements in AI raise significant ethical and societal concerns. Current AI thinking must grapple with:
- Privacy Violations: AI systems that process personal data raise concerns about data security and privacy breaches.
- Autonomous Weapons: The development of lethal autonomous weapons systems poses significant ethical challenges.
- Job Displacement: Automation driven by AI could lead to widespread job losses in various sectors.
Establishing ethical guidelines and promoting responsible AI development is crucial to mitigating these risks and ensuring AI benefits all of society. Open public discourse and robust regulatory frameworks are essential for navigating the complex ethical landscape of AI.
Conclusion
Current AI thinking reveals a field characterized by both remarkable progress and significant challenges. Understanding both the impressive capabilities and the inherent constraints of current AI systems is vital for responsible innovation. We've seen the transformative potential of AI in diverse fields, from healthcare to finance, but we must also address the ethical concerns and societal implications. Stay informed about the evolving landscape of current AI thinking to navigate the future responsibly and harness the power of AI for good. Further exploration into the ethical considerations of artificial intelligence is crucial for a future shaped by beneficial AI.
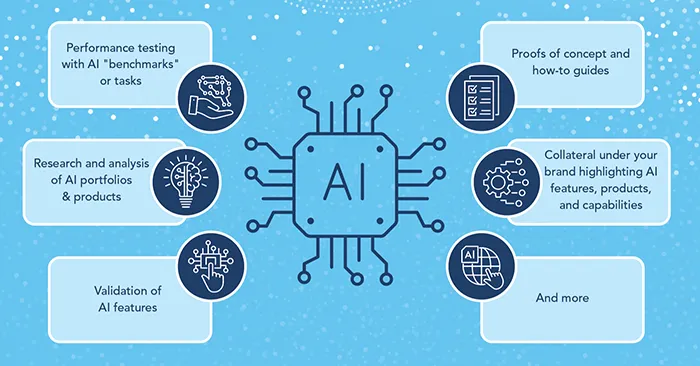
Featured Posts
-
Understanding Australian Porsche Preferences A Comparative Analysis
Apr 29, 2025 -
Uguaglianza Sul Lavoro Analisi Della Situazione E Sfide Future
Apr 29, 2025 -
Porsche 911 S T In Exclusive Riviera Blue Pts For Sale
Apr 29, 2025 -
North Korea Confirms Troop Deployment To Russia In Ukraine First Official Confirmation
Apr 29, 2025 -
Attorney General Issues Transgender Sports Ban Ultimatum To Minnesota
Apr 29, 2025