D-Wave's Quantum Computing Advancements (QBTS) Revolutionizing Drug Discovery With AI
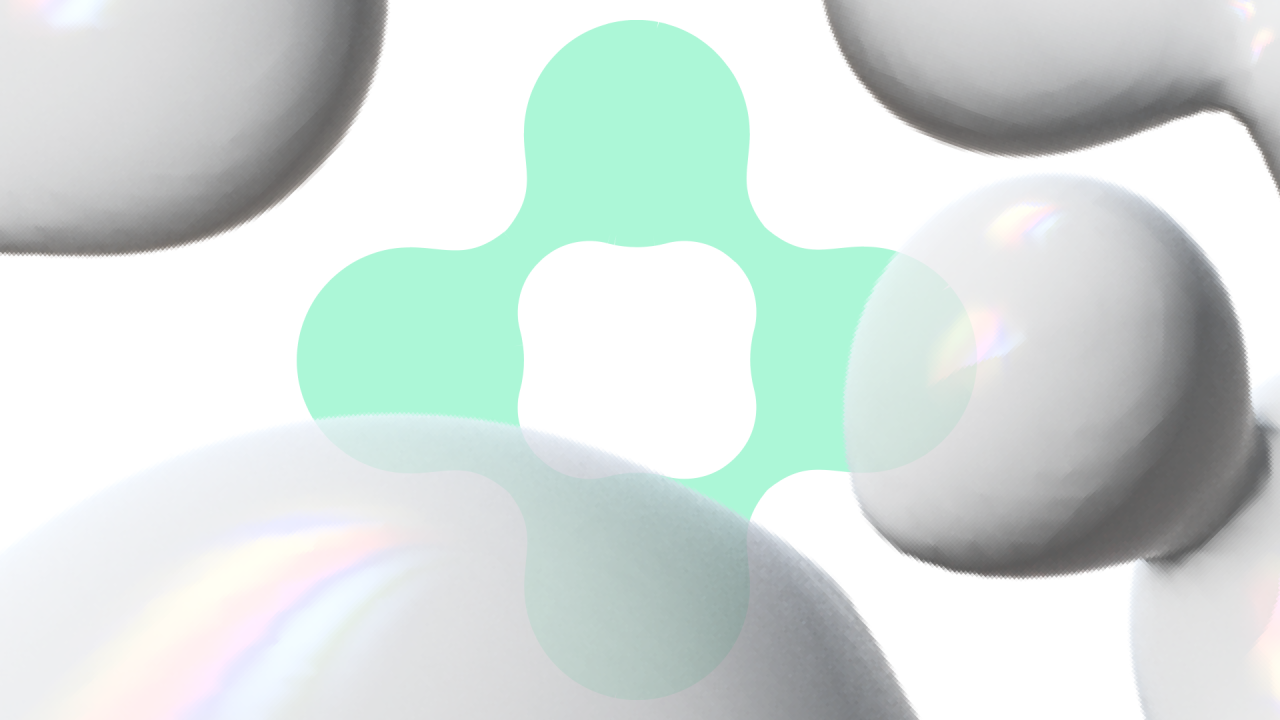
Table of Contents
D-Wave's Quantum Annealing Technology (QBTS) and its Unique Advantages
D-Wave Systems is a leader in the development of quantum annealing computers, a specialized type of quantum computer designed to solve optimization problems. Unlike gate-based quantum computers, which operate on qubits that can be manipulated in various ways, D-Wave's Quantum Annealing utilizes qubits to find the lowest energy state of a system, thus finding the optimal solution among a vast number of possibilities. This approach is particularly well-suited to the complex optimization problems prevalent in drug discovery.
The advantages of D-Wave's QBTS technology for drug discovery are compelling:
-
Faster Computation for Complex Problems: Drug design involves optimizing molecular structures for efficacy and minimizing side effects. QBTS excels at finding optimal solutions within incredibly complex chemical spaces, significantly accelerating this computationally intensive process. It does this by efficiently exploring a vast landscape of possibilities far exceeding the capabilities of classical computers.
-
Handling Large Datasets: AI-driven drug discovery relies on the analysis of massive datasets encompassing genomic information, protein structures, and clinical trial data. QBTS can handle and process these large datasets with greater speed and efficiency compared to traditional computing methods. This allows AI algorithms to train on larger datasets and generate more accurate predictions.
-
Integration with Classical Computing: D-Wave's hybrid approach combines the strengths of both quantum and classical computing. Classical computers pre-process data, design algorithms, and post-process the results from the quantum annealer, optimizing the overall workflow. This hybrid architecture maximizes the benefits of both worlds, creating a powerful tool for drug discovery.
AI's Synergistic Role in Accelerating Drug Discovery with QBTS
Artificial intelligence plays a crucial role throughout the drug discovery pipeline. AI algorithms are employed at various stages, greatly benefiting from the computational power of D-Wave's QBTS:
-
Target Identification: AI can analyze vast biological datasets to identify potential drug targets—specific proteins, genes, or pathways involved in a disease. QBTS can accelerate this process by optimizing the search for the most promising targets.
-
Lead Compound Discovery: Identifying molecules with the desired properties to interact with the target is a time-consuming process. AI algorithms, coupled with QBTS, can efficiently screen vast chemical libraries, predicting the effectiveness of potential drug candidates and identifying the most promising leads.
-
Drug Optimization: Once lead compounds are identified, AI can be used to optimize their structure, improving efficacy and reducing side effects. QBTS can contribute to this process by optimizing the molecular design for optimal binding and minimizing toxicity.
Specific AI techniques benefit significantly from QBTS:
-
Machine Learning Models: Quantum-enhanced machine learning models can be trained on larger datasets and learn more complex relationships, leading to better predictions of drug efficacy and toxicity.
-
Deep Learning Networks: Deep learning, known for its power in analyzing complex data, can be significantly accelerated using QBTS, enabling deeper insights into biological systems and drug interactions.
-
Generative Models: QBTS can enhance generative models, speeding up the creation of new drug candidates with desired properties, boosting efficiency and innovation.
Real-world Applications and Case Studies of D-Wave's QBTS in Drug Discovery
While specific details of many applications are often protected by intellectual property agreements, D-Wave is collaborating with various pharmaceutical companies and research institutions to apply its QBTS technology to drug discovery. These collaborations focus on utilizing QBTS's optimization capabilities to accelerate various stages of the drug development pipeline. For example, research suggests that QBTS could optimize the design of novel molecules, predict their properties, and enhance the speed and efficiency of clinical trials. Successful case studies are emerging, although often not publicly detailed due to the competitive nature of the pharmaceutical industry. The impact of QBTS, though still in its early stages, is expected to grow significantly as the technology advances and more collaborations mature.
Challenges and Future Directions of QBTS in Drug Discovery
Despite the considerable promise, several challenges remain:
-
Scalability: Scaling up quantum computers to handle even larger and more complex problems is an ongoing challenge. Increased qubit count and improved coherence times are crucial for future advancements.
-
Error Correction: Quantum computers are susceptible to errors. Developing more robust error correction methods is vital for improving the reliability and accuracy of QBTS in drug discovery.
-
Algorithm Development: Designing efficient quantum algorithms tailored to specific drug discovery problems is an active area of research. Further development of specialized algorithms will be essential to fully unlock the potential of QBTS.
Future advancements in QBTS and related technologies could lead to significant breakthroughs:
-
Improved qubit coherence and connectivity will increase the size and complexity of problems solvable with QBTS.
-
Advances in error correction will enhance the reliability and accuracy of the quantum computations.
-
Development of new quantum algorithms will expand the range of applications of QBTS in drug discovery.
Conclusion: The Future of Drug Discovery is Quantum: Embracing D-Wave's QBTS and AI
D-Wave's Quantum Binary Tree Search (QBTS) technology, combined with the power of artificial intelligence, offers a powerful and transformative approach to drug discovery. By accelerating computations, handling vast datasets, and optimizing complex processes, QBTS promises to significantly reduce the time and cost associated with bringing new drugs to market. The synergistic relationship between QBTS and AI is key to unlocking the full potential of this technology. The future of drug discovery is undoubtedly intertwined with quantum computing, and D-Wave's QBTS is at the forefront of this revolution. To learn more about D-Wave's QBTS and its potential applications in drug discovery and related fields, explore further resources on the D-Wave website or contact them directly to discover how D-Wave's Quantum Computing Advancements (QBTS) Revolutionizing Drug Discovery with AI can benefit your research.
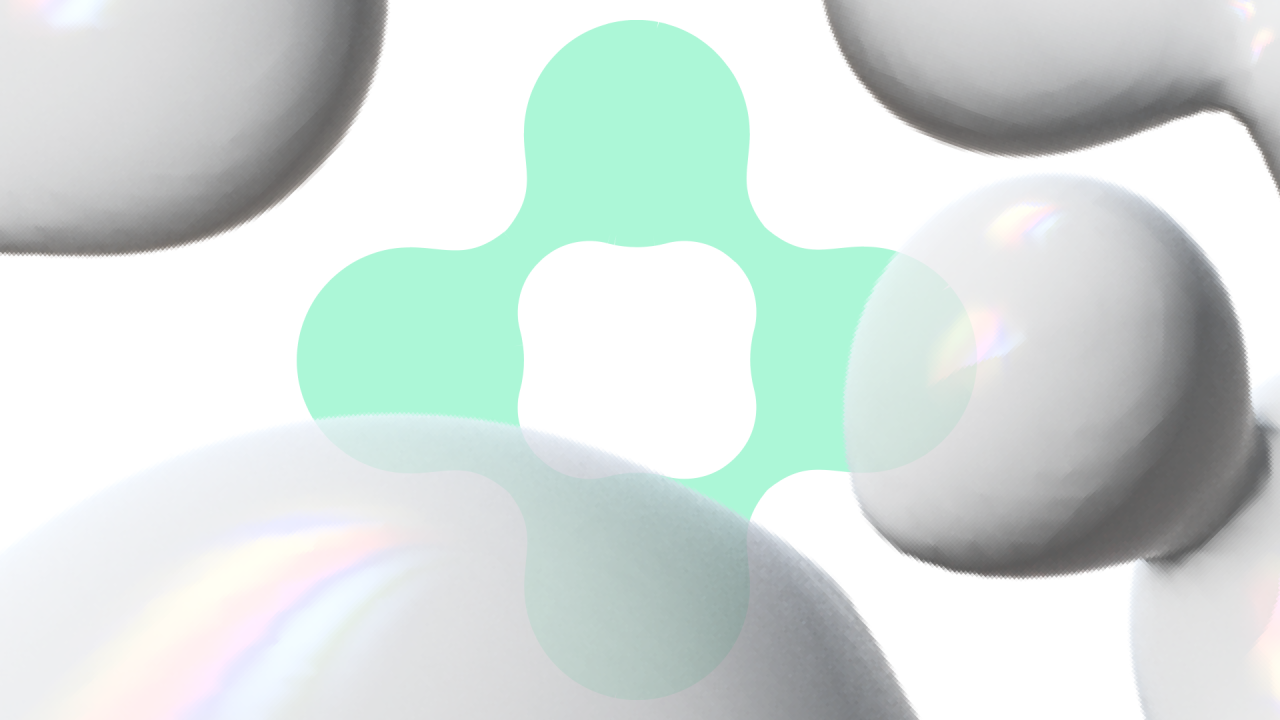
Featured Posts
-
Mothers Jail Sentence And Loss Of Home After Southport Stabbing Tweet
May 21, 2025 -
Big Bear Ai Bbai Deep Dive Into The Reasons Behind The Market Decline
May 21, 2025 -
Huuhkajataehdet Kaellman Ja Hoskonen Palaavat Suomeen
May 21, 2025 -
Rare Sighting Paulina Gretzky And Husband Step Out Together
May 21, 2025 -
Improving Siri Apples Focus On Large Language Models
May 21, 2025
Latest Posts
-
Tigers 8 Rockies 6 A Closer Look At The Upset
May 22, 2025 -
Rockies Vs Tigers An 8 6 Surprise
May 22, 2025 -
Unexpected Victory Tigers Triumph Over Rockies 8 6
May 22, 2025 -
United Kingdom Tory Wifes Imprisonment Stands Following Migrant Incident
May 22, 2025 -
Former Tory Councillors Wife Fights Racial Hatred Conviction
May 22, 2025