Exploring The Cognitive Capabilities Of Modern AI Systems
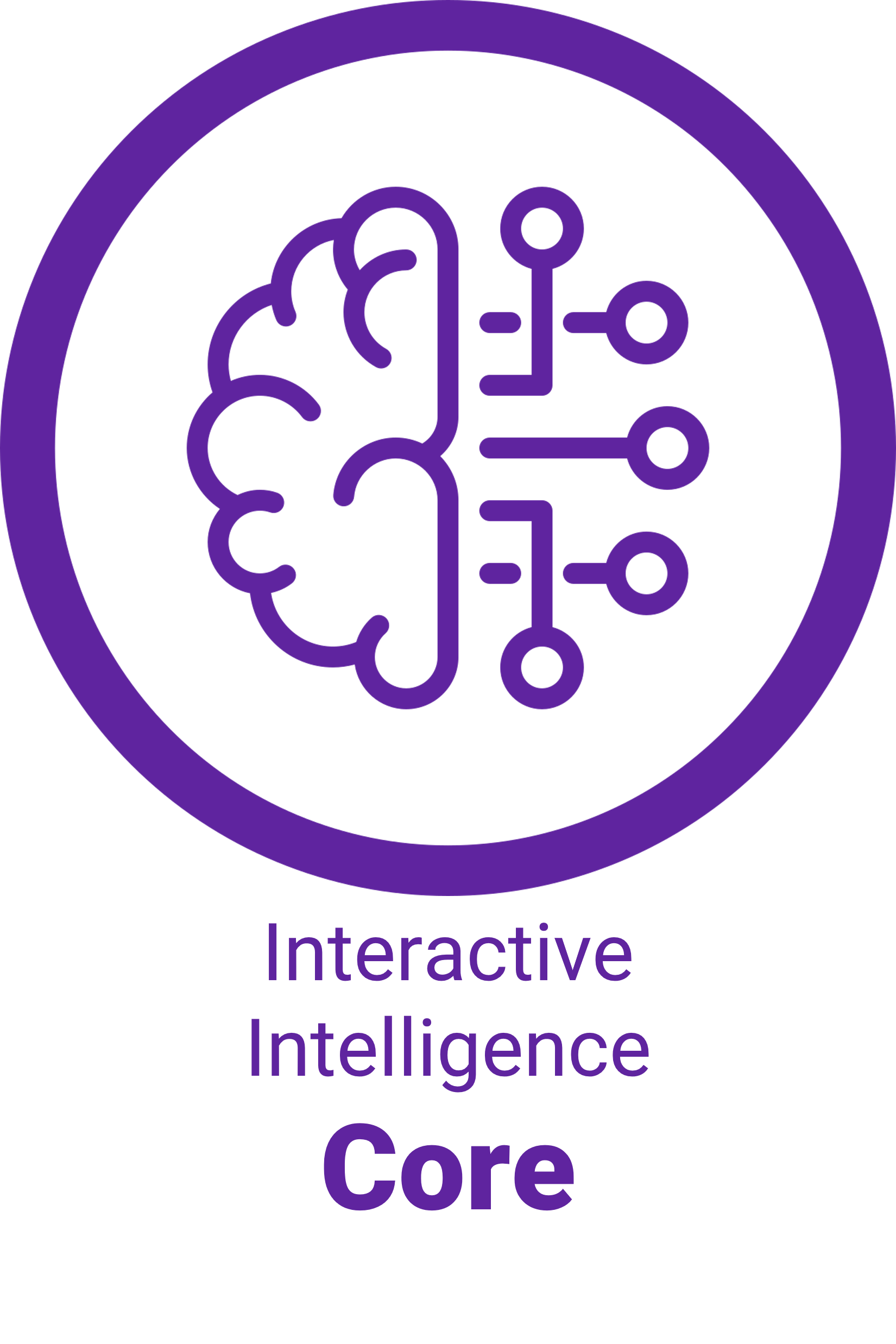
Table of Contents
Modern AI systems are rapidly evolving, exhibiting increasingly sophisticated cognitive capabilities that blur the lines between human and artificial intelligence. This article explores the remarkable advancements in AI cognition, examining its strengths, weaknesses, and potential future implications. We'll delve into key areas of AI cognitive development, providing insights into this transformative technology.
Natural Language Processing (NLP) and Understanding
The Evolution of NLP: From simple keyword matching to complex contextual understanding.
The journey of Natural Language Processing (NLP) has been remarkable. Early NLP systems relied heavily on simple keyword matching, offering limited understanding of context or nuance. However, recent advancements have propelled NLP to new heights.
- Improved sentiment analysis: AI can now accurately gauge the emotional tone of text, crucial for market research, customer service, and social media monitoring.
- Machine translation advancements: Real-time translation services, powered by deep learning, are becoming increasingly accurate and fluent.
- The rise of large language models (LLMs): Models like GPT-3 and LaMDA demonstrate unprecedented capabilities in generating human-quality text, answering questions, and even exhibiting creative writing skills.
These advancements are evident in various applications: chatbots providing personalized customer support, virtual assistants scheduling appointments, and AI-powered tools automatically generating marketing copy and summaries.
Limitations of Current NLP Systems: Challenges in handling nuances of language, ambiguity, and sarcasm.
Despite significant progress, current NLP systems still face challenges. The complexities of human language, including subtle nuances, ambiguity, and sarcasm, remain difficult for AI to fully grasp.
- Bias in training data: AI models trained on biased data can perpetuate and amplify existing societal biases in their output.
- Difficulty with context switching: Maintaining consistent context across long conversations or complex documents remains a challenge.
- Lack of genuine understanding versus pattern recognition: While AI can identify patterns and generate text that resembles human language, it doesn't necessarily possess true comprehension.
These limitations raise ethical considerations, particularly concerning the potential for misinformation and the need for responsible AI development. Addressing these challenges is crucial for the future of NLP.
Computer Vision and Image Recognition
Advances in Image Processing: Object detection, facial recognition, and image classification.
Computer vision, a field focused on enabling computers to "see," has witnessed groundbreaking advancements. AI systems can now perform complex tasks such as:
- Object detection: Identifying and locating objects within images or videos, essential for autonomous driving and security surveillance.
- Facial recognition: Identifying individuals based on their facial features, widely used in security and law enforcement.
- Image classification: Categorizing images into predefined classes, valuable in medical imaging, retail, and e-commerce.
Deep learning architectures, particularly Convolutional Neural Networks (CNNs), have revolutionized image recognition. CNNs excel at extracting meaningful features from images, leading to highly accurate and efficient image processing systems.
Challenges in Visual Cognition: Handling variations in lighting, perspective, and occlusion.
While computer vision has made remarkable progress, challenges remain in replicating the robustness and adaptability of human vision.
- The need for robust and generalizable models: AI models need to perform consistently across diverse conditions, handling variations in lighting, perspective, and occlusion (objects being partially hidden).
- The ethical considerations of facial recognition technology: Concerns about privacy, bias, and potential misuse necessitate careful consideration and regulation.
Understanding the differences between supervised and unsupervised learning methods is critical to addressing these challenges. Supervised learning requires labeled data for training, while unsupervised learning allows the AI to learn patterns from unlabeled data, potentially leading to more robust and generalizable models.
Reasoning and Problem-Solving in AI
Rule-Based Systems vs. Machine Learning: A comparison of different approaches to AI reasoning.
AI reasoning systems employ different approaches to problem-solving.
- Rule-based systems: These systems rely on predefined rules and logic to make decisions. Expert systems, designed to mimic the decision-making of human experts, are a prime example.
- Decision trees: These hierarchical structures classify data based on a series of decisions, useful for decision support and predictive modeling.
- Reinforcement learning: This approach allows AI agents to learn through trial and error, optimizing their actions to achieve a specific goal.
Each approach has its strengths and weaknesses. Rule-based systems are transparent but can be inflexible, while machine learning models can be more adaptable but lack explainability.
The Limits of Current AI Reasoning: The difficulty of handling complex, unpredictable scenarios.
Current AI systems struggle with tasks requiring common sense reasoning, causal inference, and explainability.
- Common sense reasoning: AI often lacks the intuitive understanding of the world that humans possess.
- Causal inference: Determining cause-and-effect relationships remains a significant challenge.
- Explainability: Understanding how an AI arrives at a specific decision is crucial for trust and accountability.
Ongoing research focuses on improving AI reasoning capabilities through advancements in knowledge representation, reasoning architectures, and explainable AI (XAI) techniques.
Conclusion
Modern AI systems have made remarkable strides in cognitive capabilities, demonstrating impressive advancements in NLP, computer vision, and reasoning. However, significant challenges remain in achieving true human-level cognition. Areas like common sense reasoning, explainability, and ethical considerations require further research and development.
To stay informed about the exciting developments in the field of AI cognitive capabilities, continue exploring resources and research in artificial intelligence. Understanding the strengths and limitations of modern AI systems is crucial for navigating the future of this transformative technology. Further exploration into the cognitive capabilities of modern AI is essential to harness its potential responsibly.
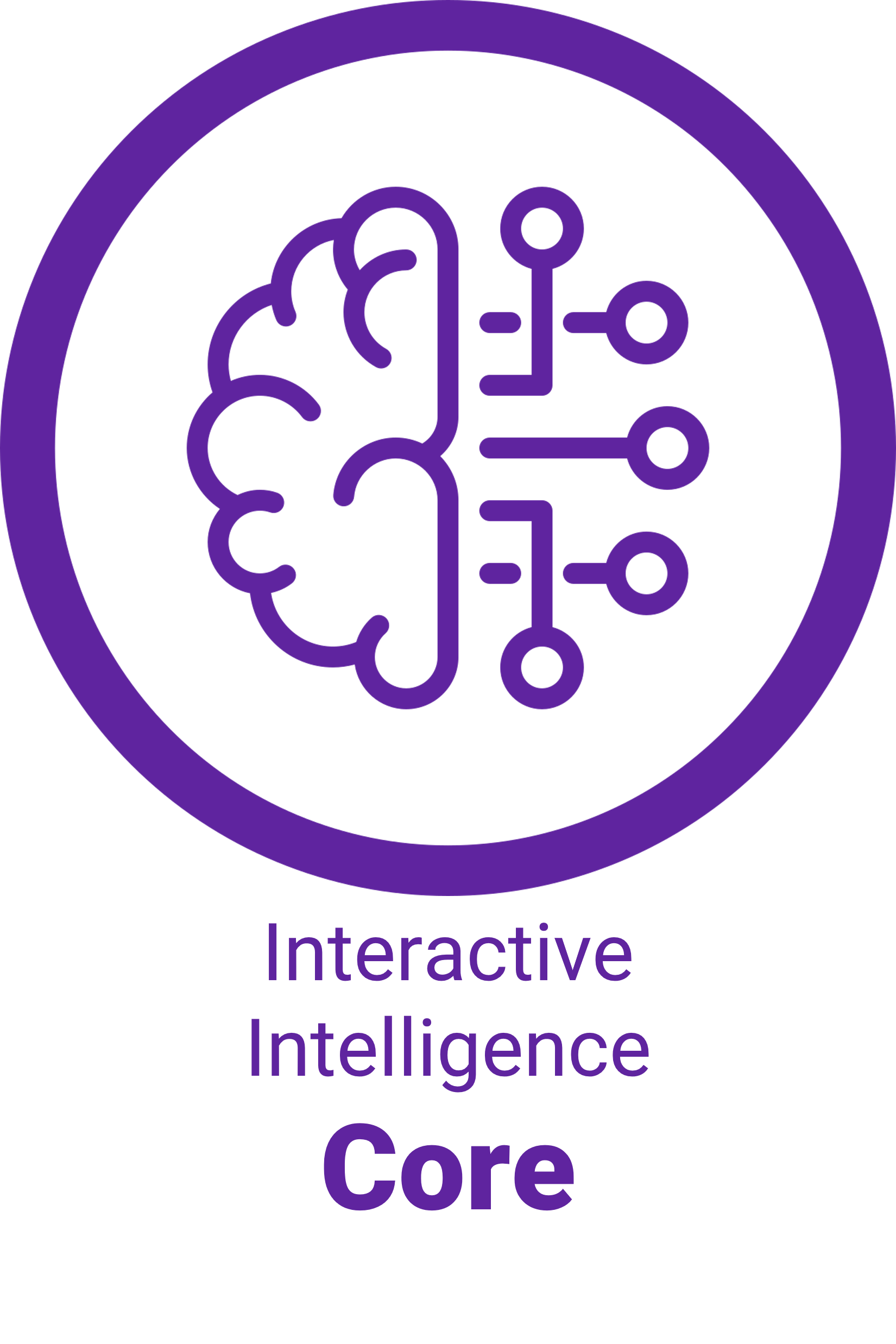
Featured Posts
-
Improved Mail Service Predicted For Louisville Following Delays
Apr 29, 2025 -
Spy Photos Hint At 2026 Porsche Cayenne Ev Design
Apr 29, 2025 -
5 Key Dos And Don Ts To Secure A Private Credit Role
Apr 29, 2025 -
U S Dollars Bleak Outlook Worst Start Since Nixon
Apr 29, 2025 -
Retail Sales Slump Fuels Rate Cut Predictions From Economists
Apr 29, 2025