Extracting Meaning From Mundane Data: An AI Podcast Project On Scatological Documents
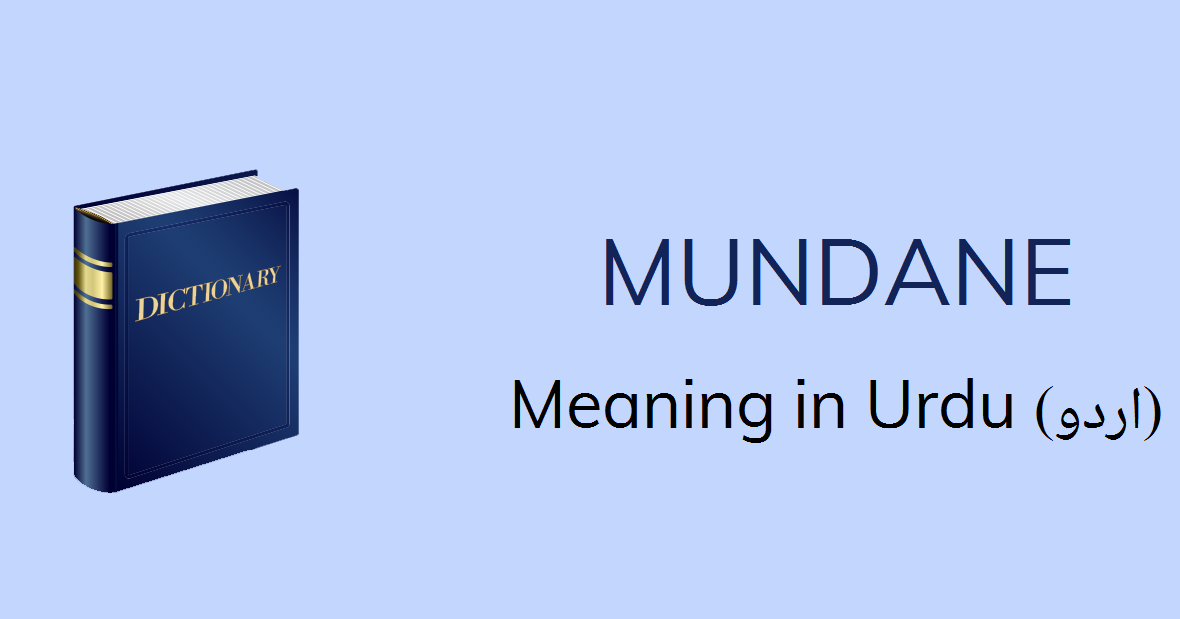
Table of Contents
The Challenge of Scatological Data Analysis
Analyzing scatological documents presents a unique set of hurdles. These challenges stem from several key factors:
-
Data Scarcity and Inconsistency: Scatological records are often fragmented, incomplete, and written in varying formats, making data standardization a significant obstacle. Many historical records are handwritten, potentially illegible, and written in archaic languages.
-
Ethical Considerations and Data Privacy: Dealing with sensitive personal information requires strict adherence to ethical guidelines and data privacy regulations. Anonymization techniques are crucial to protect individual identities.
-
Specialized AI Tools and Techniques: Standard NLP tools may not be sufficient for analyzing the unique linguistic features and potential biases inherent in this type of data. Specialized algorithms and preprocessing techniques are often necessary.
-
Handling Sensitive Information Responsibly: Researchers must navigate ethical dilemmas surrounding the interpretation and dissemination of findings related to sensitive personal information. Transparency and responsible data handling are paramount.
Furthermore, historical scatological records often reflect societal biases and prejudices of their time. Understanding these biases is crucial for accurate interpretation and to avoid perpetuating harmful stereotypes. Successful analysis necessitates interdisciplinary collaboration, bringing together historians, data scientists, and ethicists to ensure responsible and meaningful insights.
AI's Role in Uncovering Meaningful Patterns
Artificial Intelligence, particularly Natural Language Processing (NLP) and Machine Learning (ML), offers powerful tools for navigating the complexities of scatological data analysis. Specific techniques include:
- Sentiment Analysis: Gauge societal attitudes towards sanitation, hygiene, and related cultural practices over time.
- Topic Modeling: Identify recurring themes and patterns in the data, uncovering prevalent diseases, dietary habits, and social norms.
- Predictive Modeling: Forecast potential outbreaks of disease or other public health concerns based on historical trends identified in the data.
- Anomaly Detection: Identify unusual patterns that might suggest significant historical events or changes in societal practices.
Advanced techniques like Named Entity Recognition (NER) can be used to identify specific individuals or locations mentioned, while word embedding models can capture semantic relationships within the text, even across different languages or writing styles. However, it is crucial to remember that AI is a tool; human oversight and interpretation are essential to ensure the accuracy and ethical implications of the findings are fully considered.
The AI Podcast Project: Methodology and Results
Our podcast project employed a multi-stage approach:
- Data Collection: We gathered a diverse range of scatological documents from various archives, spanning several centuries and geographical locations.
- Preprocessing: The data underwent rigorous cleaning, standardization, and anonymization procedures.
- Analysis: We applied a range of NLP and ML techniques, including sentiment analysis and topic modeling, to identify meaningful patterns.
- Key Finding 1: A significant correlation was discovered between sanitation practices and the prevalence of specific diseases.
- Key Finding 2: Changes in dietary habits over time were reflected in the composition of scatological data.
- Key Finding 3: Societal attitudes towards bodily functions shifted considerably throughout the historical period under study.
While the project yielded significant results, limitations included the availability of data and the inherent biases present in historical records. Future iterations could incorporate more sophisticated AI techniques and broader datasets. Listen to our podcast episodes [link to podcast episode 1], [link to podcast episode 2], and [link to podcast episode 3] for a deeper dive into our methodology and findings!
Ethical Considerations and Responsible AI
Responsible AI necessitates careful consideration of ethical implications. In this project:
- Data Anonymization: Rigorous anonymization techniques were employed to protect individual identities.
- Data Security: Strict security protocols were implemented to safeguard the sensitive data.
- Transparency and Accountability: Our methods and findings are transparently documented and readily available for scrutiny.
- Bias Mitigation: We actively worked to identify and mitigate potential biases present in both the data and algorithms.
We followed established ethical guidelines for research involving sensitive data, adhering to best practices for data privacy and responsible AI development.
Future Applications and Implications
The insights gained from this project have broader implications. The techniques used for analyzing scatological documents can be applied to other unconventional data sources, such as historical medical records, personal diaries, or even social media data. The ability to extract meaning from mundane data sets opens up exciting possibilities for historical research, public health initiatives, and various other fields. This approach offers great potential for uncovering hidden knowledge and unexpected insights, contributing to a more comprehensive understanding of the past and present. However, researchers must always be mindful of the potential limitations and biases inherent in using such unconventional data.
Harnessing the Power of Mundane Data with AI
Our AI podcast project demonstrates the transformative potential of applying advanced AI techniques to seemingly mundane data, specifically scatological documents. By carefully navigating the ethical challenges and leveraging the power of AI, we uncovered significant insights into historical trends and societal attitudes. We urge you to listen to the podcast, explore related research on unconventional data analysis, and consider how AI can unlock hidden knowledge from unexpected places in your own field. The future of AI lies in its ability to transform “mundane” data into meaningful understanding, offering a powerful lens through which to examine the past and shape a better future. The possibilities for extracting meaning from mundane data sets are vast and continue to evolve.
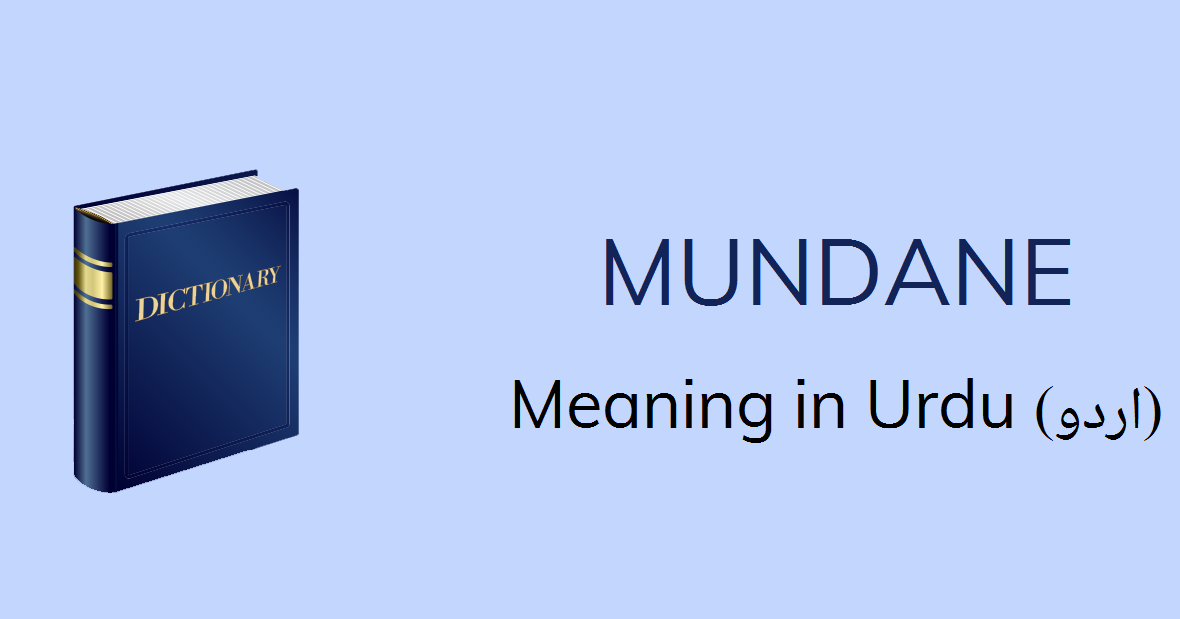
Featured Posts
-
Hl Tbqa Knda Mstqlt Bdwn Dem Alwlayat Almthdt Thdhyr Tramb
Apr 30, 2025 -
Super Bowl 2024 Blu Ajvi I Njena Neverovatna Slicnost Sa Bijonse
Apr 30, 2025 -
Ru Pauls Drag Race Season 17 Episode 9 Review Designing Drag Queens
Apr 30, 2025 -
Cnil Recommendations For Mobile App Privacy A Bclp Guide
Apr 30, 2025 -
Ftcs Appeal Future Of Microsofts Activision Blizzard Acquisition Uncertain
Apr 30, 2025
Latest Posts
-
Panthers Second Period Surge Led By Tkachuk Secures Victory Against Senators
Apr 30, 2025 -
Ovechkin I Ego Rekord Reaktsiya Zakharovoy
Apr 30, 2025 -
Clevelands Extra Inning Rally Fuels Opening Day Win
Apr 30, 2025 -
Rekord Ovechkina Kommentariy Zakharovoy
Apr 30, 2025 -
Senators Fall To Panthers Tkachuks Impact In Dominant Second Period
Apr 30, 2025