How AI Processes Information: A Deep Dive Into Artificial Thinking
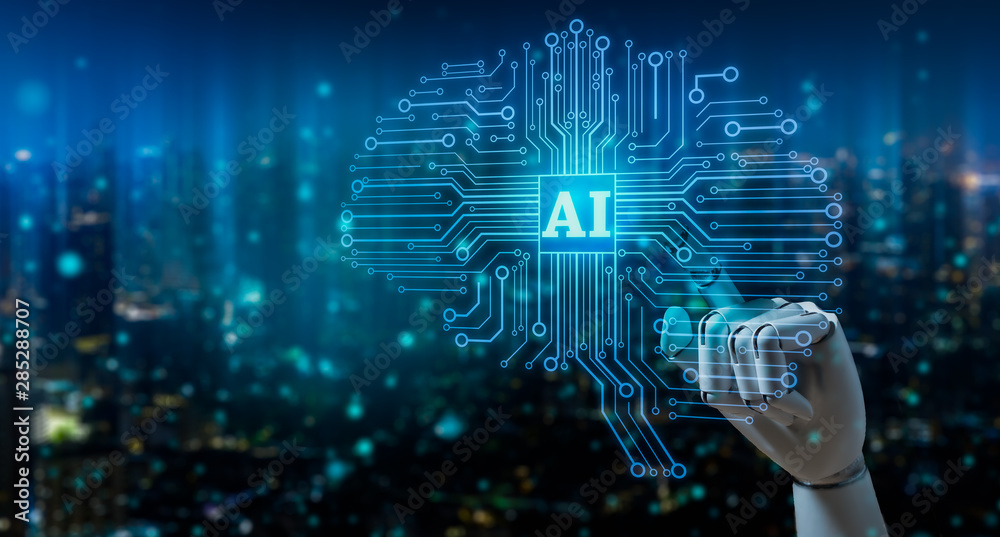
Table of Contents
Data Input and Preprocessing: The Foundation of AI Thinking
High-quality data is the bedrock of any successful AI system. Garbage in, garbage out – this adage is especially true for AI. The accuracy and effectiveness of an AI model are directly proportional to the quality of the data it's trained on. AI systems process various data formats, including:
- Text: Used for natural language processing (NLP) tasks like sentiment analysis, machine translation, and chatbot development.
- Images: Crucial for computer vision applications such as object recognition, image classification, and medical image analysis.
- Audio: Essential for speech recognition, voice assistants, and music generation.
- Video: Used in video analysis, surveillance systems, and autonomous driving.
Before this data can be used, however, it needs preprocessing. This involves several crucial steps:
- Data cleaning: This critical stage handles missing values (imputation techniques like mean/median imputation), outliers (removal or transformation), and inconsistencies (data standardization and normalization).
- Data transformation: This step involves scaling (e.g., min-max scaling, standardization) and normalization to bring data into a consistent range, and encoding categorical variables (e.g., one-hot encoding) to convert them into numerical representations suitable for algorithms.
- Feature extraction: This process selects the most relevant features from the raw data to improve model performance and reduce dimensionality. This can involve techniques like principal component analysis (PCA) or feature selection algorithms.
Algorithms and Models: The Engine of AI Processing
AI algorithms are the "brains" of the operation, determining how the system learns and makes decisions. Different types of algorithms are suited for different tasks:
- Supervised learning: Models learn from labeled data, where each data point is tagged with the correct output. Examples include linear regression, support vector machines (SVMs), and decision trees, used for tasks like image classification and spam detection.
- Unsupervised learning: Models identify patterns and structures in unlabeled data. Techniques like clustering (k-means, hierarchical clustering) and dimensionality reduction (PCA) are used for tasks like customer segmentation and anomaly detection.
- Reinforcement learning: Models learn through trial and error, interacting with an environment and receiving rewards or penalties for their actions. This is used for applications like robotics, game playing (AlphaGo), and resource management.
Popular AI models include:
- Neural networks: Inspired by the human brain, these models consist of interconnected nodes (neurons) that process information. Deep learning, a subset of machine learning, utilizes deep neural networks with multiple layers to extract complex features.
- Decision trees: These models use a tree-like structure to make decisions based on a series of rules. They're easy to interpret and visualize.
- Support vector machines (SVMs): These models find the optimal hyperplane that separates different classes of data. They're particularly effective in high-dimensional spaces.
Learning and Adaptation: How AI Improves Over Time
Machine learning is the core of how AI systems improve over time. It's the process of enabling computers to learn from data without explicit programming. This learning process typically involves:
- Training: The model is fed large amounts of data, and its internal parameters are adjusted to minimize errors and improve accuracy. This often involves optimization algorithms like gradient descent and backpropagation.
- Validation: The trained model is evaluated on a separate dataset (validation set) to assess its performance on unseen data. This helps prevent overfitting, where the model performs well on training data but poorly on new data.
- Testing: A final evaluation on a held-out test set provides an unbiased estimate of the model's generalization ability – its capacity to perform well on completely new, unseen data.
Output and Interpretation: Understanding AI's Results
AI models generate output in various forms, including predictions, classifications, and recommendations. However, understanding and interpreting these results is crucial. This includes:
- Understanding confidence levels and probabilities: AI models often provide probabilities or confidence scores associated with their predictions. These indicate the certainty of the model's output.
- Identifying potential sources of bias: AI models can inherit biases present in the data they are trained on, leading to unfair or discriminatory outcomes. Careful data selection and model evaluation are vital to mitigate this risk.
- The role of human oversight: Human experts are needed to interpret AI results, validate findings, and ensure ethical and responsible deployment. AI should be seen as a tool to augment human capabilities, not replace them entirely.
Conclusion: Mastering the Art of AI Information Processing
Understanding how AI processes information—from data input and preprocessing to algorithm selection, learning, and output interpretation—is vital for anyone seeking to leverage this powerful technology. The responsible development and deployment of AI require a deep understanding of its mechanisms and limitations. By acknowledging the potential biases and incorporating human oversight, we can harness the power of AI for good. Want to delve deeper into the intricacies of how AI processes information? Explore our other resources on [link to relevant resource] to expand your understanding of artificial thinking and its impact on the future. The evolution of AI is ongoing, and continuous learning about this transformative technology is crucial.
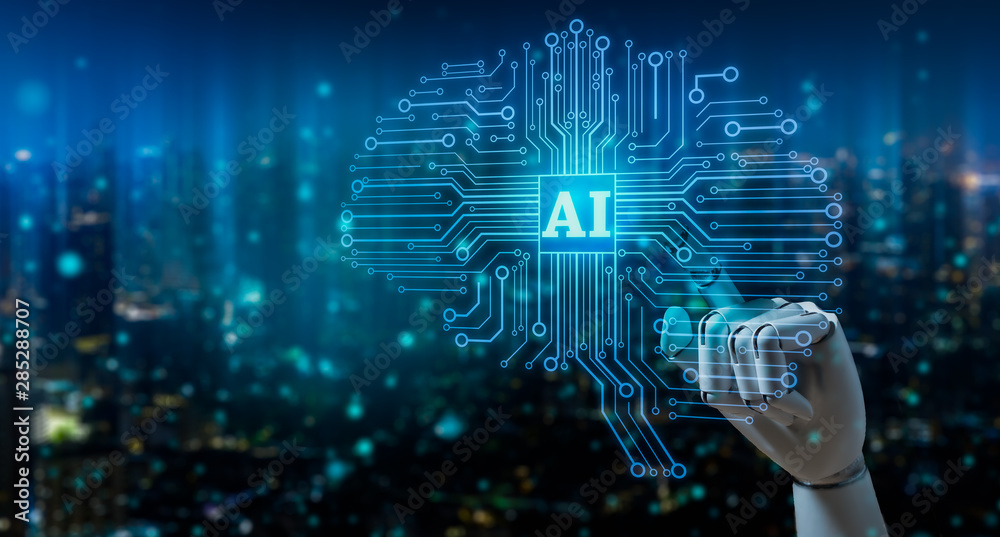
Featured Posts
-
V Mware Costs To Skyrocket At And T Reports 1050 Price Increase From Broadcom
Apr 29, 2025 -
Can Film Tax Credits Boost Minnesotas Tv And Film Industry
Apr 29, 2025 -
154 Albums And Counting Willie Nelsons Latest Release And Family News
Apr 29, 2025 -
Nyt Spelling Bee March 13 2025 Find The Pangram And All Answers
Apr 29, 2025 -
Beyond Quinoa Discover The Latest Health Food Sensation
Apr 29, 2025
Latest Posts
-
Solve Nyt Strands Hints For Puzzle 393 March 31
Apr 29, 2025 -
Nyt Spelling Bee April 3 2025 Find The Pangram And All Answers
Apr 29, 2025 -
Nyt Strands Solutions Hints And Answers For March 3 2025
Apr 29, 2025 -
Solve The Nyt Spelling Bee February 28 2025 Puzzle
Apr 29, 2025 -
Nyt Spelling Bee April 3 2025 Clues Answers And Pangram
Apr 29, 2025