Improving The Accuracy Of Automated Visual Inspection Systems For Lyophilized Vials
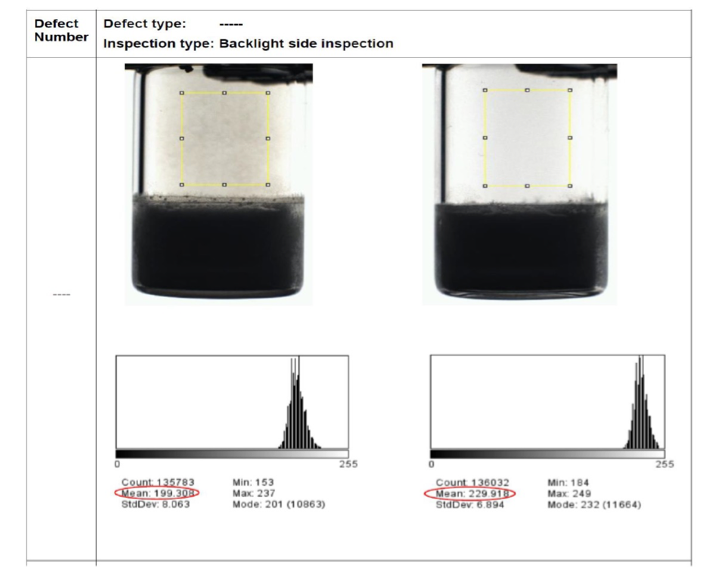
Table of Contents
Advanced Imaging Techniques for Enhanced Defect Detection
Traditional methods, such as human visual inspection, are inherently limited. Human inspectors can experience fatigue, leading to missed defects, and subjective interpretations can cause inconsistencies. Automated systems, however, can be equipped with advanced imaging techniques to overcome these limitations and significantly improve defect detection.
High-Resolution Imaging
High-resolution cameras coupled with advanced lighting techniques like structured lighting and polarized light are crucial for enhancing defect visibility. These improvements provide:
- Improved detection of subtle cracks, particulate matter, and other defects: High resolution allows for the identification of even the smallest imperfections that might be missed by the naked eye or lower-resolution systems.
- Reduced false positives and negatives: Sharper images lead to more accurate defect classification, minimizing errors.
- Enhanced image clarity for easier analysis by AI algorithms: Superior image quality provides better input for machine learning algorithms, improving their accuracy and efficiency in identifying defects.
Multispectral Imaging
Moving beyond visible light, multispectral or hyperspectral imaging offers a significant advantage. These techniques capture images across a wider range of wavelengths, revealing information invisible to standard cameras:
- Detection of subtle color variations or chemical changes indicating defects: Multispectral imaging can detect variations in color or chemical composition that are indicative of defects, even if they are not visually apparent.
- Improved discrimination between defects and benign variations in appearance: The additional spectral information helps to differentiate between genuine defects and harmless variations in the appearance of the lyophilized product.
- Enhanced capability for detecting contaminants: Multispectral imaging can be particularly effective in identifying contaminants that may be difficult to detect using visible light alone.
3D Imaging
For comprehensive surface analysis, 3D imaging techniques such as structured light 3D scanning provide unparalleled detail:
- Accurate measurement of vial dimensions and surface irregularities: 3D imaging allows for precise measurements of vial dimensions and the detection of even minute surface irregularities.
- Improved detection of defects on curved surfaces: Unlike 2D imaging, 3D scanning captures the entire vial surface, enabling the detection of defects on curved areas.
- Creation of detailed 3D models for defect analysis: The resulting 3D models allow for detailed analysis of defects, providing valuable insights into their nature and origin.
Artificial Intelligence (AI) and Machine Learning (ML) for Improved Accuracy
AI and ML algorithms are revolutionizing automated visual inspection. These powerful tools can analyze image data with incredible speed and accuracy, far surpassing human capabilities.
Deep Learning for Defect Classification
Deep learning models, a subset of machine learning, are particularly well-suited for defect classification in lyophilized vials:
- High accuracy in defect detection even with complex variations in vial appearance: Deep learning models can learn to recognize a wide range of defects, even those that exhibit significant variations in appearance.
- Ability to learn and adapt to new defect types over time: These models can be continuously retrained with new data, allowing them to adapt to emerging defect types and improve their accuracy over time.
- Reduced need for manual intervention in the inspection process: Deep learning significantly reduces the need for human intervention, increasing efficiency and reducing the risk of human error.
Data Augmentation and Model Training
The success of AI-powered inspection systems relies heavily on the quality and quantity of training data. Data augmentation plays a critical role:
- Generating synthetic data to supplement real-world data: Generating synthetic data can expand the training dataset, especially when real-world defect data is limited.
- Using data augmentation techniques to increase the diversity of the training dataset: Techniques like rotation, scaling, and noise addition can improve the robustness and generalizability of the AI models.
- Regular model retraining to maintain accuracy and adapt to changing conditions: Regular retraining with updated data ensures that the AI models remain accurate and adapt to any changes in the manufacturing process or defect types.
System Calibration and Validation for Consistent Performance
Maintaining the accuracy of automated visual inspection systems requires rigorous calibration and validation procedures. These steps are essential for ensuring consistent performance and compliance with regulatory standards.
Regular Calibration Procedures
Regular calibration is vital to maintain accuracy:
- Using calibrated standards and reference materials: Calibration should be performed using standardized materials with known defect characteristics.
- Establishing clear calibration procedures and documentation: Detailed procedures and documentation are essential to ensure consistency and traceability.
- Tracking calibration data and results: Calibration data should be meticulously tracked and analyzed to identify any trends or issues.
Validation and Verification
Validation demonstrates that the system meets its intended performance requirements:
- Conducting performance qualification (PQ) and operational qualification (OQ): PQ and OQ are critical steps in validating the system’s performance and ensuring it operates as intended.
- Demonstrating compliance with relevant regulatory guidelines (e.g., GMP): Compliance with Good Manufacturing Practices (GMP) and other regulatory guidelines is crucial for ensuring product safety and quality.
- Implementing a robust quality control program: A comprehensive quality control program ensures the ongoing accuracy and reliability of the inspection system.
Conclusion
Improving the accuracy of automated visual inspection systems for lyophilized vials is crucial for ensuring product quality and patient safety in the pharmaceutical industry. By implementing advanced imaging techniques, leveraging the power of AI and ML, and adhering to rigorous calibration and validation protocols, manufacturers can significantly enhance the accuracy and efficiency of their inspection processes. Investing in and optimizing automated visual inspection systems for lyophilized vials leads to reduced costs, improved throughput, and enhanced confidence in product quality. Don't compromise on quality; explore the latest advancements in automated visual inspection systems for lyophilized vials today.
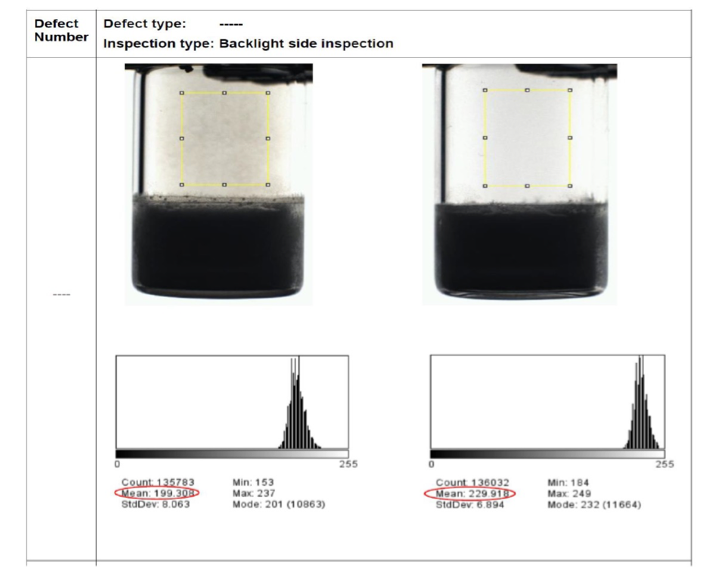
Featured Posts
-
Het Einde Van Een Era Thomas Muellers Afscheid Van Bayern Muenchen
May 11, 2025 -
Cody Bellingers Batting Order Placement And Its Impact On Aaron Judge
May 11, 2025 -
Exploring City Name A Top College Town In Michigan
May 11, 2025 -
Predicting Aaron Judges 2024 Performance Yankees Magazine Analysis
May 11, 2025 -
From Cabin Crew To Cockpit One Womans Transition From Flight Attendant To Pilot
May 11, 2025
Latest Posts
-
New James O Keefe Investigation The Latest On Prince Andrew
May 12, 2025 -
Payton Pritchards New Shoe Deal With Converse A Celtics Success Story
May 12, 2025 -
Car Crash Involving Prince Andrew Accuser Virginia Giuffre Latest Updates
May 12, 2025 -
Bus Crash Leaves Prince Andrew Accuser Fighting For Life
May 12, 2025 -
James O Keefes Undercover Scoop A Blow To Prince Andrews Reputation
May 12, 2025