Palantir Vs. The Competition: Predicting Top Performing Stocks In 3 Years
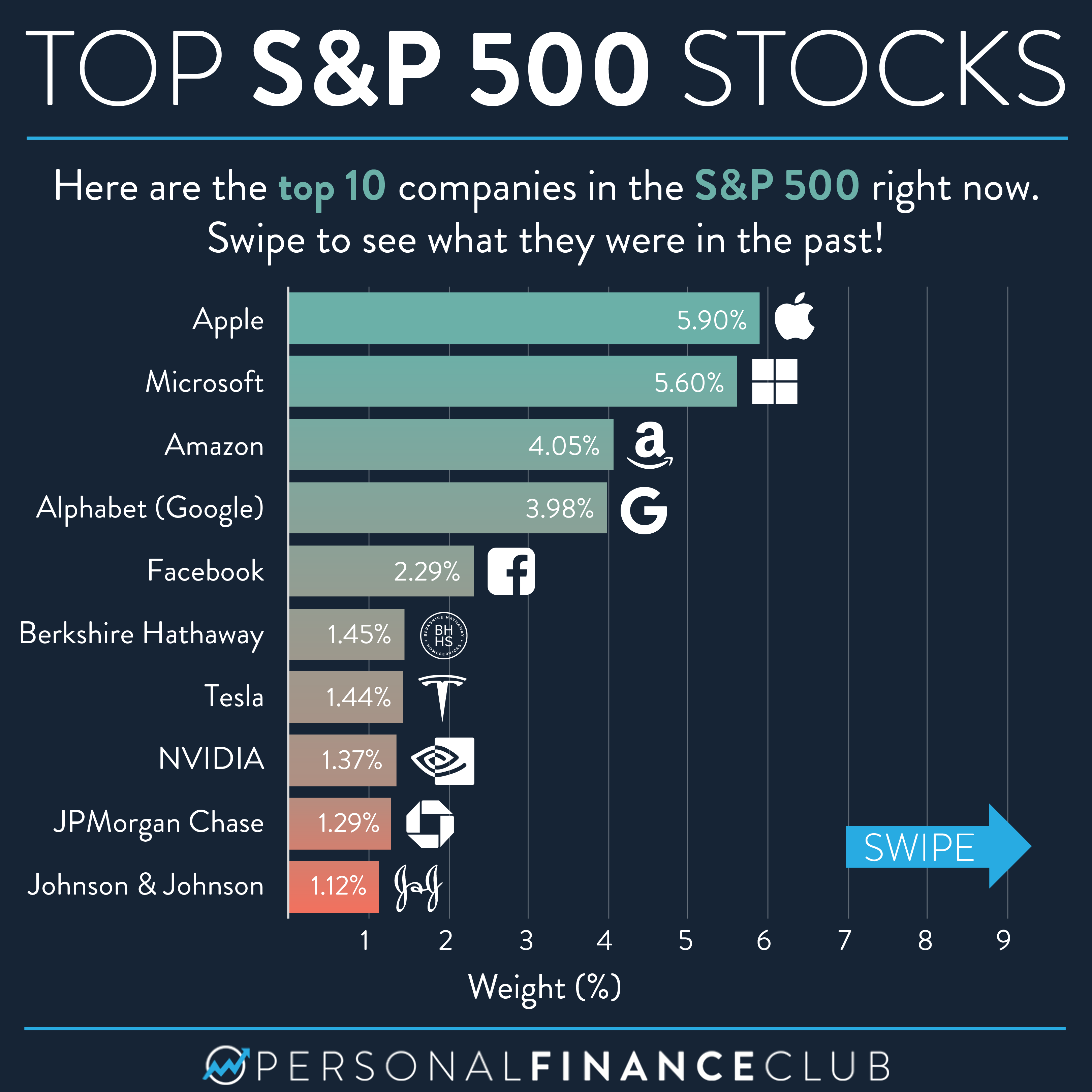
Table of Contents
Predicting the stock market's future is notoriously difficult. Only a small percentage of investors consistently achieve high returns, highlighting the immense challenge in accurately forecasting top-performing stocks. Yet, the potential rewards are significant, driving the constant search for better predictive tools and strategies. Palantir, a leading data analytics company, offers a powerful platform leveraging advanced technologies to tackle this challenge. This article compares Palantir's capabilities with those of its competitors in predicting top-performing stocks over a three-year horizon, exploring the strengths and weaknesses of various approaches to stock market prediction.
<h2>Palantir's Predictive Capabilities for Stock Market Analysis</h2>
Palantir's strength lies in its ability to integrate and analyze vast quantities of data, far exceeding the capabilities of traditional methods. This allows for a more nuanced and comprehensive approach to predicting stock performance.
<h3>Data Sources and Integration</h3>
Palantir's Foundry platform integrates diverse datasets crucial for accurate financial forecasting. This includes:
- Financial News Data: Real-time news feeds, press releases, and financial reports from reputable sources.
- Social Media Sentiment: Analysis of social media trends and public opinion regarding specific companies and sectors.
- Regulatory Filings: Access to SEC filings (10-K, 10-Q, etc.) and other regulatory disclosures.
- Alternative Data: Data from sources not traditionally used in financial modeling, such as satellite imagery, supply chain data, and web scraping results.
Palantir's Foundry acts as a central hub, enabling seamless integration and analysis of these disparate data sources, fostering a more holistic understanding of market dynamics.
<h3>Advanced Analytics and Machine Learning Algorithms</h3>
Palantir utilizes sophisticated machine learning algorithms and AI capabilities for predictive modeling, going beyond traditional financial models. These include:
- Time Series Analysis: Forecasting future stock prices based on historical patterns and trends.
- Deep Learning: Identifying complex, non-linear relationships within the data to improve prediction accuracy.
- Natural Language Processing (NLP): Extracting insights from textual data sources like news articles and social media posts.
These algorithms work together to identify potential market trends, uncover hidden correlations, and pinpoint stocks poised for significant growth over a three-year period.
<h3>Case Studies and Success Stories</h3>
While specific case studies with quantifiable results often remain confidential due to client agreements, Palantir's track record in other data-intensive fields suggests its predictive capabilities are substantial. Anecdotal evidence points to successful predictions in various sectors, highlighting the platform's potential for identifying high-growth stocks. Further research into Palantir's published case studies (where available) could reveal more concrete examples of successful stock market predictions and associated returns.
<h2>Key Competitors and Their Approaches</h2>
Palantir faces competition from several established players and emerging fintech companies in the predictive analytics space.
<h3>Traditional Financial Modeling Firms</h3>
Large investment banks and financial modeling firms have long relied on quantitative models. However, their approaches often rely heavily on:
- Historical Data: Primarily using past performance to predict future results.
- Limited Data Sources: Focusing mostly on readily available financial data and neglecting alternative data sources.
These limitations can hinder their ability to accurately predict future stock performance, especially in rapidly changing markets.
<h3>Fintech Companies with Predictive Analytics</h3>
Numerous fintech startups employ AI and ML for financial forecasting, using different data sources and algorithms. A direct comparison of their performance against Palantir's is difficult due to a lack of public benchmarks. However, some key differentiators may include:
- Data Specialization: Some fintech companies may focus on specific sectors or asset classes, potentially leading to higher accuracy within their niche.
- Algorithm Differences: Different algorithms might excel in different market conditions, creating varying levels of prediction accuracy.
<h3>Quantitative Hedge Funds</h3>
Quantitative hedge funds employ sophisticated algorithms and models for trading decisions. While they often use similar technologies to Palantir, their focus differs:
- Short-Term Trading: Many quantitative funds prioritize short-term gains rather than long-term stock predictions.
- Proprietary Strategies: Their specific algorithms and data sources are typically confidential.
Thus, a direct comparison with Palantir's longer-term focus is complex.
<h2>Challenges and Limitations of Stock Market Prediction</h2>
Despite the advancements in AI and machine learning, stock market prediction remains inherently challenging.
<h3>Market Volatility and Unpredictability</h3>
The stock market is susceptible to unpredictable events:
- Geopolitical Events: Wars, political instability, and international relations can significantly impact market performance.
- Economic Crises: Recessions, inflation, and unexpected economic shocks can cause dramatic market fluctuations.
Even the most sophisticated algorithms struggle to predict these "Black Swan" events, highlighting the inherent limitations of any predictive model.
<h3>Data Bias and Model Accuracy</h3>
Data bias can significantly affect model accuracy:
- Data Quality: Inaccurate or incomplete data can lead to flawed predictions.
- Model Validation: Thorough testing and validation are essential to ensure the model's reliability.
Addressing data bias and ensuring model accuracy are continuous challenges in stock market prediction.
<h3>Ethical Considerations</h3>
The use of AI in finance raises ethical concerns:
- Market Manipulation: The potential for using AI to manipulate market prices requires careful consideration and regulation.
- Insider Trading: The risk of using privileged information obtained through AI needs strict ethical safeguards.
Responsible development and application of AI in finance are paramount to mitigate these risks.
<h2>Conclusion: Palantir vs. The Competition: The Future of Stock Market Prediction</h2>
Palantir's platform offers a powerful approach to stock market prediction, leveraging advanced analytics and extensive data integration. However, it faces strong competition from established financial institutions and agile fintech companies. The inherent volatility and unpredictability of the market underscore the limitations of any predictive model, regardless of its sophistication. While Palantir's strengths in data integration and AI offer potential advantages, accuracy remains a challenge for all players. To learn more about Palantir and the future of stock market prediction, further research into its capabilities and the evolving landscape of predicting top-performing stocks is recommended. Explore the potential of advanced analytics in investment strategies and how tools like Palantir are shaping the future of financial forecasting.
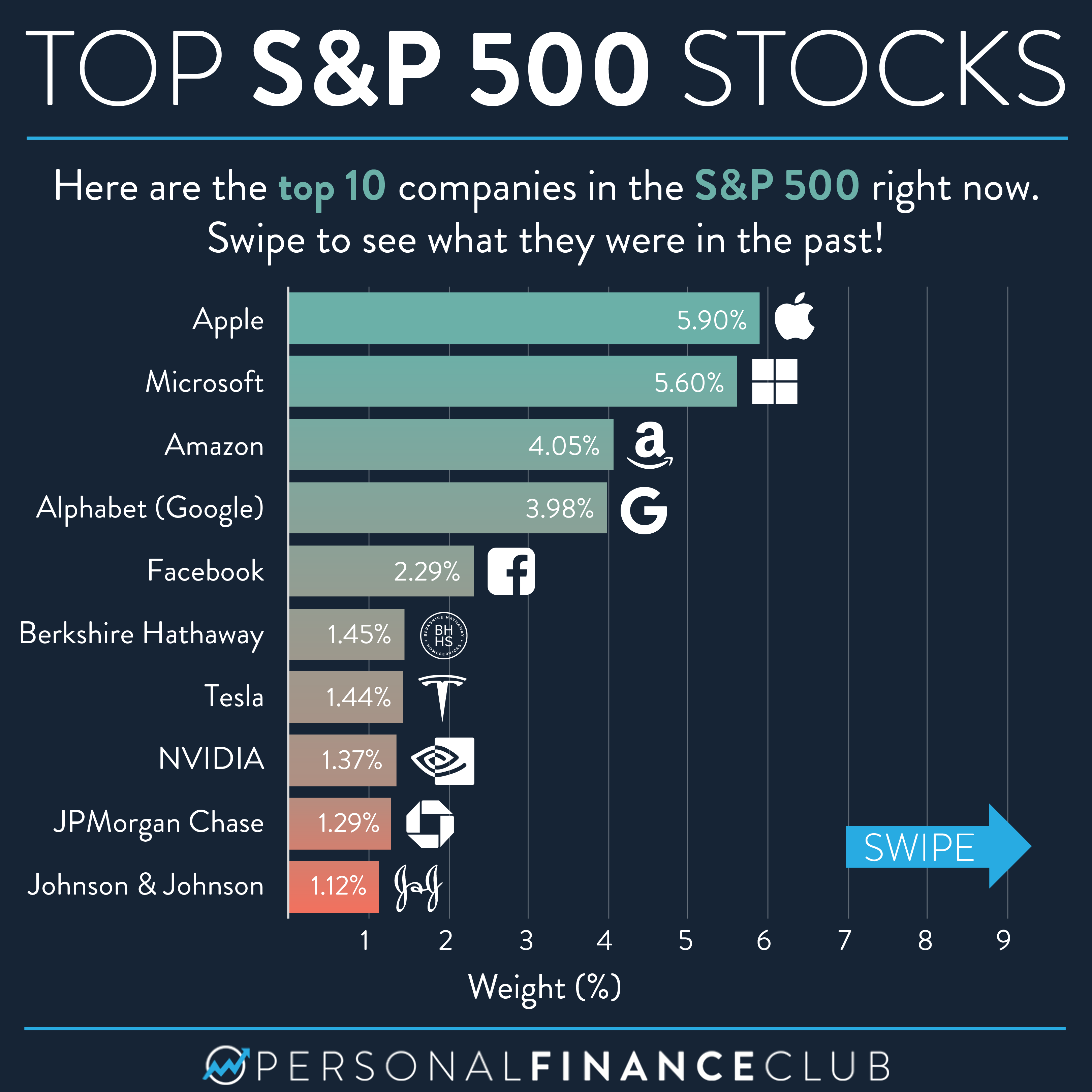
Featured Posts
-
Un Debut D Incendie Mobilise Les Pompiers A La Mediatheque Champollion De Dijon
May 09, 2025 -
Impact Of Hertl Injury On Vegas Golden Knights Playoff Run
May 09, 2025 -
2025 Bitcoin Conference Seoul Asias Bitcoin Hub
May 09, 2025 -
Whittier Shows Solidarity American Samoan Family Awaits First Court Appearance On Voter Fraud Charges
May 09, 2025 -
Money Talks Fox News Host Counters Colleagues Trump Tariff View
May 09, 2025
Latest Posts
-
Frantsiya I Polsha Podpisanie Vazhnogo Dogovora Eksklyuziv Ot Unian
May 09, 2025 -
Ukrainskiy Aspekt Oboronnogo Soglasheniya Makrona I Tuska 9 Maya
May 09, 2025 -
Dogovor Makrona I Tuska Frantsiya I Polsha Ukreplyayut Sotrudnichestvo Unian
May 09, 2025 -
Unian Novye Detali O Dogovore Mezhdu Frantsiey I Polshey Makron I Tusk
May 09, 2025 -
9 Maya Makron I Tusk Podpishut Oboronnoe Soglashenie Analiz Klyuchevykh Punktov
May 09, 2025