Podcast Creation: Using AI To Process Repetitive Scatological Documents
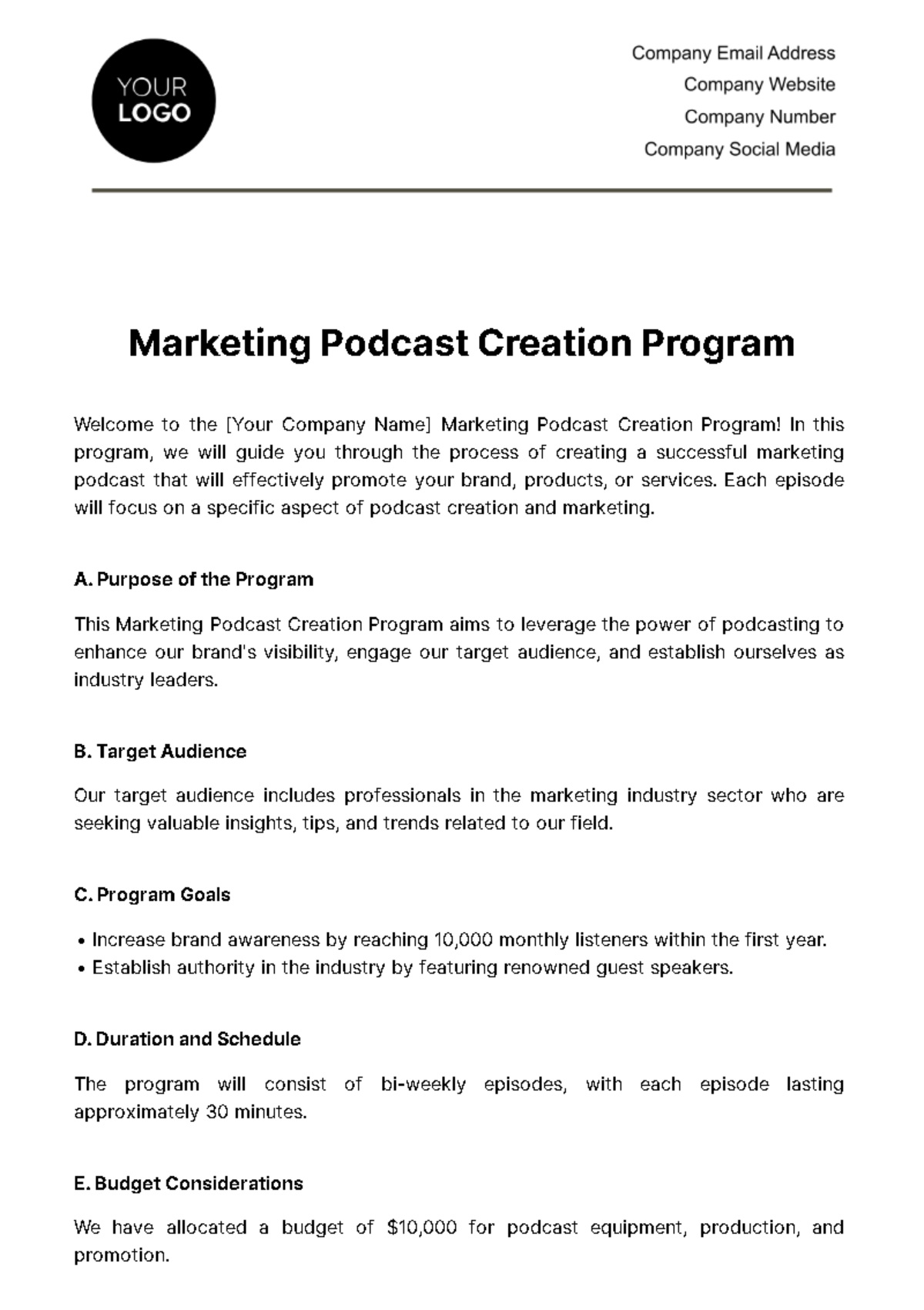
Table of Contents
Identifying the Problem: The Scatological Data Challenge in Podcast Production
Many podcasts delve into controversial or historically sensitive topics, leading to the unavoidable challenge of handling scatological data. This might include:
- Transcripts requiring extensive cleaning: Raw transcripts often contain profanity, offensive language, and irrelevant details needing meticulous removal.
- Research involving unsavory historical accounts: Researching certain historical periods or events can expose podcasters to copious amounts of graphic and unpleasant material.
- User-generated content moderation: Podcasts relying on audience submissions might encounter offensive or inappropriate comments needing filtering.
Manual processing of this type of data is incredibly time-consuming and unpleasant:
- Manual review is slow and prone to errors: Human error is inevitable during tedious tasks, potentially leading to inconsistencies or missed offensive content.
- It impacts productivity and creativity: Time spent cleaning data is time not spent on the creative aspects of podcast production – writing scripts, recording audio, editing, and marketing.
- It can lead to burnout among podcast production teams: The repetitive and often unpleasant nature of the work can be demoralizing and lead to team burnout.
AI-Powered Solutions: Automating Scatological Data Processing
Fortunately, advancements in artificial intelligence offer powerful solutions for automating the processing of scatological data. Several AI tools and techniques can significantly streamline this workflow:
- Natural Language Processing (NLP): NLP algorithms excel at cleaning and analyzing text. They can identify and filter profanity, offensive language, and irrelevant information, significantly reducing manual effort. Tools like Google Cloud Natural Language API or Amazon Comprehend can be utilized for this purpose.
- Custom Machine Learning Models: For podcasters dealing with highly specific types of scatological data, creating custom machine learning models can provide tailored solutions. This allows for more precise filtering and analysis based on specific needs and context.
- AI-powered transcription services: Some transcription services offer advanced features like profanity filtering and automated redaction, making the initial data cleaning process much more efficient.
These tools automate tasks such as:
-
Identifying and filtering out irrelevant or offensive content: AI can accurately flag and remove unwanted words, phrases, or even entire sections of text based on pre-defined rules or machine learning models.
-
Cleaning up transcripts to remove excessive profanity or graphic descriptions: AI can replace offensive words with asterisks or other placeholders, or even suggest more appropriate alternatives.
-
Summarizing large volumes of scatological data: AI can condense large amounts of data into concise summaries, highlighting key information while removing unnecessary details.
-
Specific examples of AI tools: Google Cloud Natural Language API, Amazon Comprehend, various custom-built NLP models.
-
Benefits of using each tool: Cost savings from reduced labor, increased time efficiency allowing for more creative work, improved accuracy in content filtering.
-
Ethical considerations: Careful consideration must be given to the ethical implications of using AI to filter sensitive content. Establishing clear guidelines and ensuring transparency are crucial.
Implementing AI in Your Podcast Workflow: A Step-by-Step Guide
Integrating AI into your podcast creation workflow involves several key steps:
- Data Preparation: Gather and clean your initial data. This might involve transcribing audio or organizing existing text documents.
- Model Selection: Choose an appropriate AI tool or model based on your specific needs and budget. Consider pre-trained models or custom model development.
- Model Training (if necessary): If using a custom model, this step involves training the model on a representative dataset to achieve the desired accuracy.
- Deployment: Integrate the chosen AI tool into your workflow, potentially using APIs or other integration methods.
- Model Evaluation: Monitor the model's performance and make adjustments as needed. Continuously evaluate and improve the accuracy and efficiency of the AI tool.
- Iterative Improvement: Regularly refine the AI model or chosen tools based on feedback and performance metrics.
- Step-by-step instructions: Detailed guides on specific AI tools are readily available online through their respective documentation.
- Tips for troubleshooting common issues: Troubleshooting guides can usually be found within the chosen tool's support documentation or online forums.
- Resources for finding and using AI tools: Explore cloud-based AI services (AWS, Google Cloud, Azure) and open-source NLP libraries.
Case Studies: Successful AI Implementation in Podcast Production
While specific examples of podcasts publicly showcasing AI usage for scatological data processing are currently limited (due to the sensitive nature of the data), the principles remain highly relevant. Imagine a historical podcast processing thousands of pages of primary source materials, many containing offensive language. Utilizing AI to filter and summarize this data would drastically reduce processing time and allow researchers to focus on analysis and narrative development. The potential cost savings and time gains are substantial.
- Specific examples (hypothetical): A true crime podcast cleaning transcripts of police interviews, a history podcast analyzing letters from war correspondents.
- Quantifiable results (hypothetical): A 50% reduction in processing time, a 20% decrease in production costs.
- Lessons learned (hypothetical): Careful data preparation is crucial for accurate AI processing; continuous monitoring and refinement are vital for optimal performance.
Conclusion: Streamlining Podcast Creation with AI for Scatological Data
In conclusion, AI offers a powerful solution for streamlining podcast creation by significantly improving the efficiency and reducing the burden of processing repetitive scatological data. The benefits are undeniable: time savings, cost-effectiveness, improved accuracy, and a greatly reduced workload, freeing podcasters to focus on the creative elements of their work. Start using AI for your podcast creation today, and transform your podcast workflow with AI-powered scatological data processing. Don't let tedious data cleaning hold back your podcasting success.
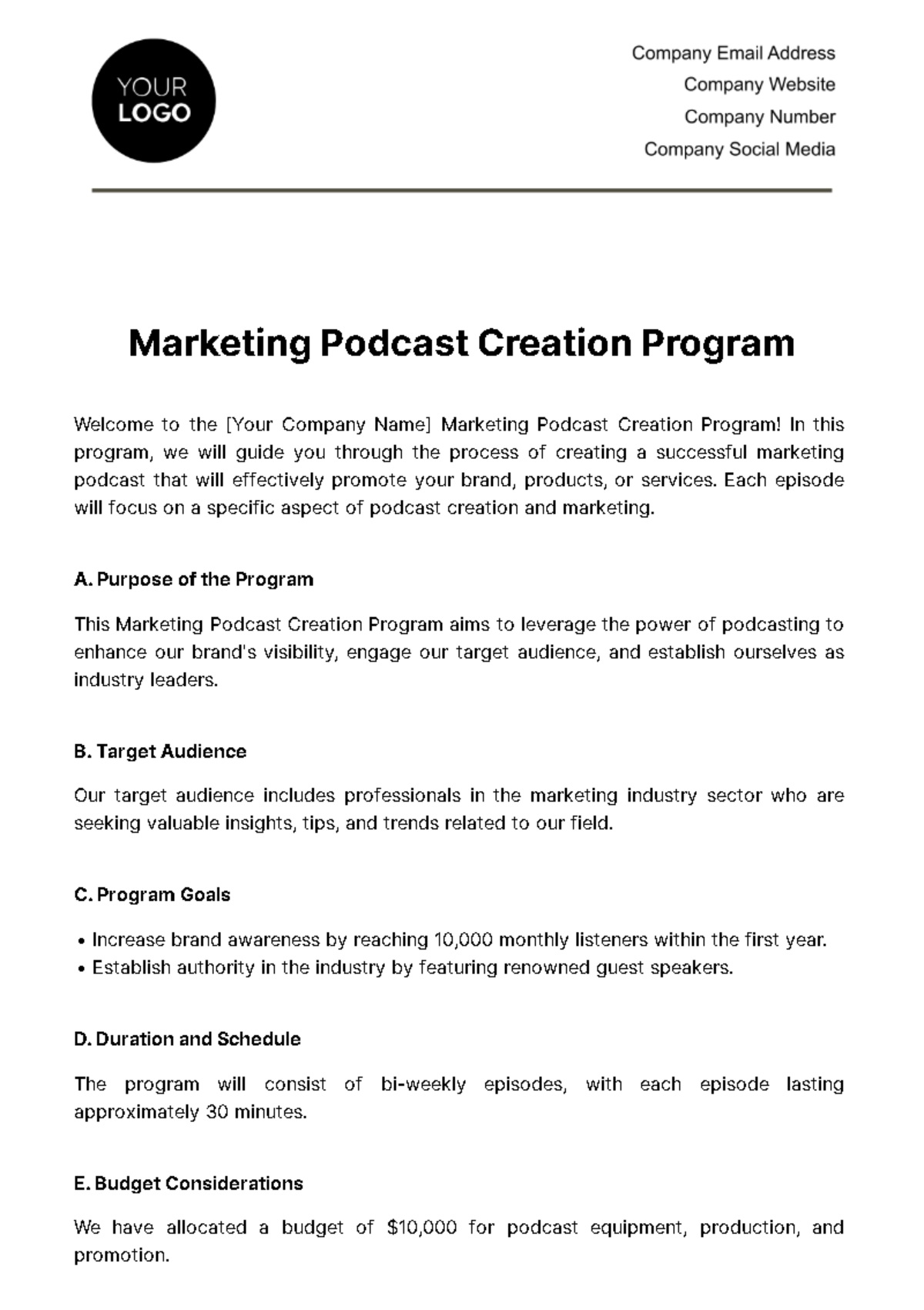
Featured Posts
-
Harvards Future A Conservative Professors Plan For Reform
Apr 26, 2025 -
Analyzing Shedeur Sanders Nfl Draft Stock An Espn Analysts Deep Dive
Apr 26, 2025 -
Tour De France Vingegaards Road To Recovery From Concussion
Apr 26, 2025 -
Shedeur Sanders Nfl Draft Potential An Espn Analysts Perspective
Apr 26, 2025 -
Are Trumps Tariffs Harming The Economy Ceos Share Their Concerns
Apr 26, 2025
Latest Posts
-
Will Ahmed Hassanein Break Barriers In The Nfl Draft
Apr 26, 2025 -
The Ahmed Hassanein Story From Egypt To The Nfl
Apr 26, 2025 -
Ahmed Hassaneins Improbable Journey To The Nfl Draft
Apr 26, 2025 -
Nfl Draft 2024 First Round In Green Bay
Apr 26, 2025 -
The Karen Read Trials A Chronological Overview
Apr 26, 2025