Repetitive Scatological Documents: AI's Role In Transforming Data Into A "Poop" Podcast
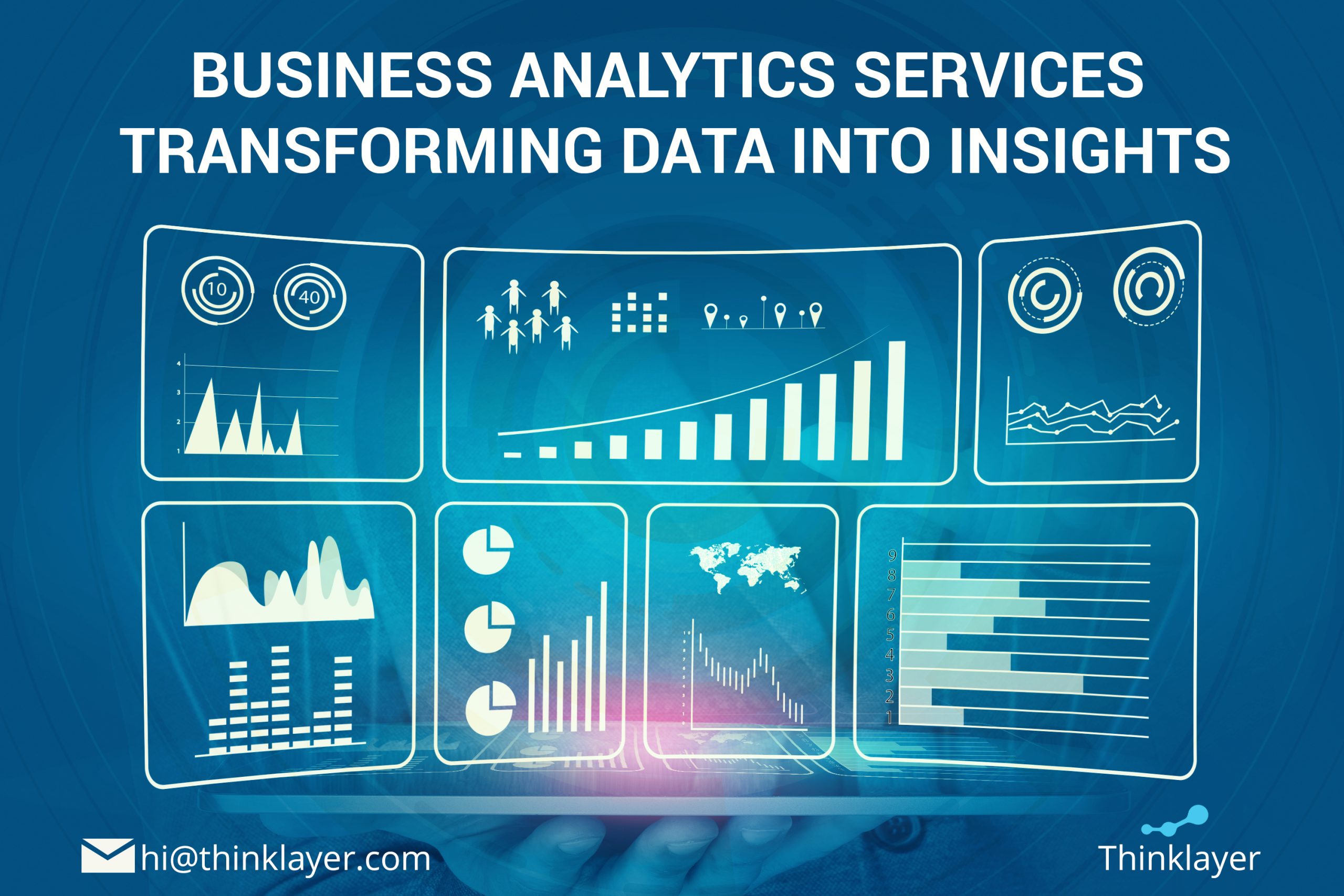
Table of Contents
The Challenge of Repetitive Scatological Data Analysis
Analyzing scatological data, while crucial for various fields, presents significant challenges. The sheer volume and repetitive nature of this data often overwhelm traditional methods.
Data Volume and Redundancy
The volume of repetitive scatological data can be staggering. Consider:
- Medical records: Thousands of entries detailing bowel movements, stool consistency, and other relevant information.
- Research studies: Extensive datasets from clinical trials investigating digestive health and related conditions.
- Environmental monitoring: Data on fecal contamination in water sources, requiring meticulous analysis for public health assessments.
Manually analyzing such data is incredibly time-consuming, prone to human error, and often inefficient. The redundancy makes it difficult to extract meaningful insights without significant preprocessing.
Data Cleaning and Preprocessing
Before AI algorithms can effectively analyze scatological data, rigorous cleaning and preprocessing are essential. This crucial step involves:
- Noise reduction: Removing irrelevant or erroneous data points.
- Outlier detection: Identifying and handling unusual data points that might skew results.
- Data normalization: Transforming data into a consistent format for easier analysis.
Accurate data is paramount for reliable AI-driven insights. Without proper cleaning, the results will be compromised, leading to inaccurate conclusions and potentially harmful interpretations.
Traditional Methods vs. AI
Traditional manual analysis of repetitive scatological documents is slow, labor-intensive, and susceptible to bias. AI offers several advantages:
- Faster processing: AI algorithms can process vast datasets significantly faster than humans.
- Higher accuracy: AI reduces human error, leading to more reliable results.
- Pattern identification: AI can identify subtle patterns and correlations invisible to the human eye, uncovering hidden insights.
AI's Role in Transforming Scatological Data into Podcast Content
AI's capabilities are transforming how we handle and interpret scatological data, opening up exciting opportunities for podcast creation.
Natural Language Processing (NLP)
NLP is a powerful AI technique that extracts meaningful information from text-based scatological data. Key NLP tasks include:
- Topic modeling: Identifying recurring themes and topics within the data.
- Sentiment analysis: Determining the emotional tone of descriptions (e.g., positive, negative, neutral).
- Named entity recognition: Identifying and classifying key entities like medications, diseases, or geographical locations.
NLP allows for the identification of trends and insights that might otherwise be missed during manual analysis, providing rich material for podcast episodes.
Machine Learning for Pattern Recognition
Machine learning algorithms can identify complex patterns and correlations within scatological data. Techniques like:
- Clustering: Grouping similar data points together to reveal distinct patterns.
- Classification: Categorizing data points into predefined categories (e.g., different types of bowel movements).
These patterns form the backbone of engaging podcast narratives, offering listeners insights into the complexities of digestive health, environmental impacts, or medical research findings.
Data Visualization and Storytelling
To create truly compelling podcast narratives, data visualization is crucial. Transforming complex data into easily digestible formats is key. Consider:
- Charts and graphs: Visually representing trends and correlations.
- Interactive elements: Engaging listeners with dynamic data presentations.
The goal is to translate complex data into engaging stories, making the information accessible and captivating to a broader audience.
Ethical Considerations and Privacy Concerns
Handling sensitive scatological data requires careful attention to ethical considerations and privacy protection.
Data Anonymization and Privacy
Protecting patient/subject privacy is paramount. Essential steps include:
- Data de-identification: Removing identifying information to prevent individual identification.
- Encryption: Protecting data using strong encryption methods during storage and transmission.
Strict adherence to regulations like HIPAA (in the US) and GDPR (in Europe) is crucial.
Responsible AI Development and Deployment
Responsible AI development is key to avoiding bias and ensuring ethical data usage. This involves:
- Bias detection: Identifying and mitigating potential biases in AI models.
- Transparency: Ensuring that the data processing and AI model are transparent and understandable.
The Future of "Poop" Podcasts – Powered by AI
AI's ability to transform repetitive scatological documents into valuable and engaging podcast content is revolutionizing how we understand and discuss this often-overlooked area of data. AI offers speed, accuracy, the ability to uncover hidden patterns, and the power to create compelling narratives. Start transforming your repetitive scatological documents into engaging podcasts today! The power of AI is waiting to be unleashed.
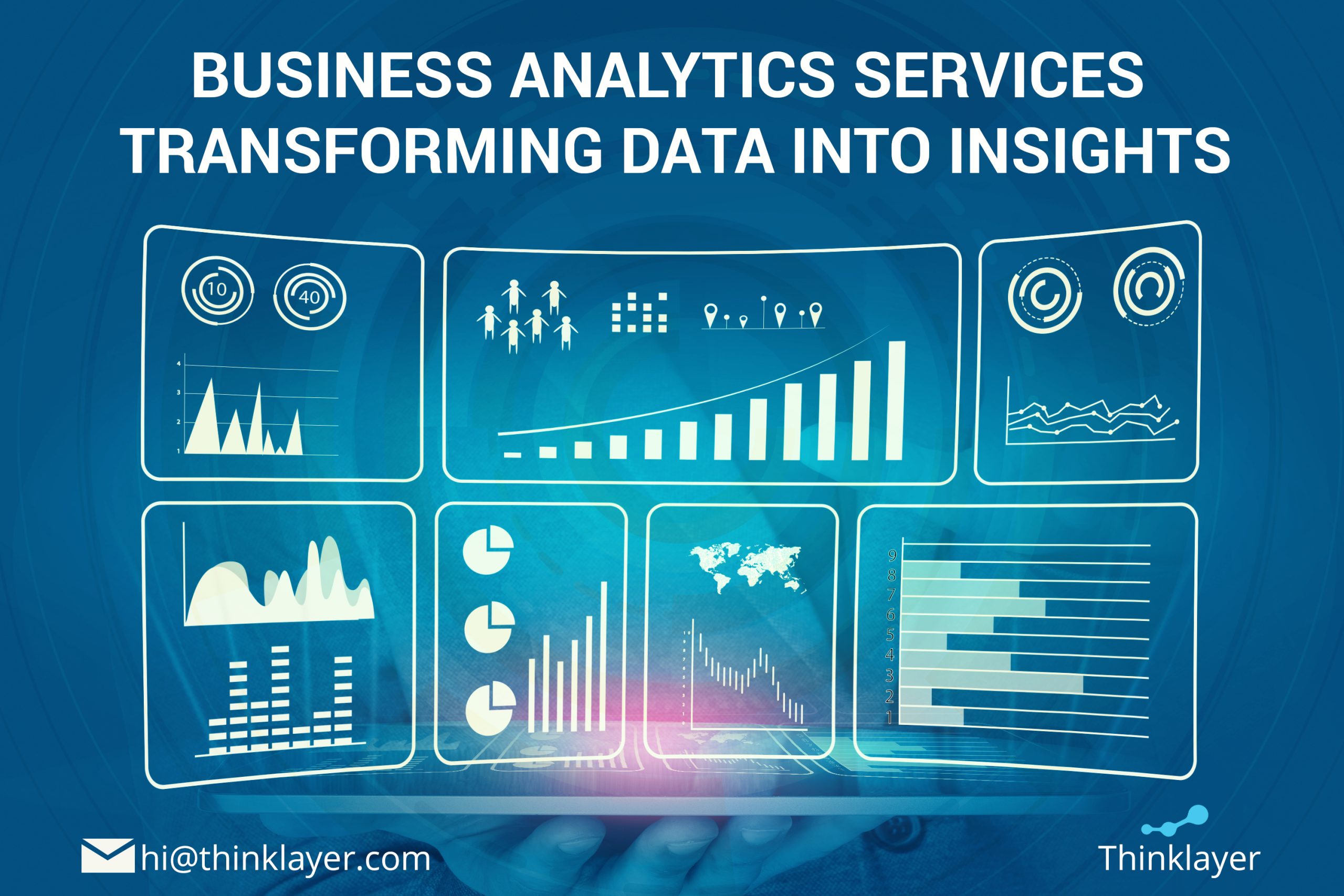
Featured Posts
-
The Blaugrana Journey Of Ramiro Helmeyer
Apr 27, 2025 -
Securing A Nintendo Switch 2 My Game Stop Preorder Journey
Apr 27, 2025 -
Microsofts Vision A Design Chiefs Perspective On Ais Impact On Humanity
Apr 27, 2025 -
El Exito En El Gol Con La Metodologia De Alberto Ardila Olivares
Apr 27, 2025 -
What To Do On A Happy Day February 20 2025
Apr 27, 2025
Latest Posts
-
Impacto De La Eliminacion De Paolini Y Pegula En El Wta 1000 De Dubai
Apr 27, 2025 -
Resultados Wta 1000 Dubai Caida De Favoritas Como Paolini Y Pegula
Apr 27, 2025 -
Despedida Temprana Para Paolini Y Pegula En El Wta 1000 De Dubai
Apr 27, 2025 -
Wta 1000 Dubai Analisis De La Derrota De Paolini Y Pegula
Apr 27, 2025 -
Sorpresivas Eliminaciones En Wta 1000 Dubai Paolini Y Pegula
Apr 27, 2025