The Illusion Of AI Intelligence: Debunking Common Misconceptions
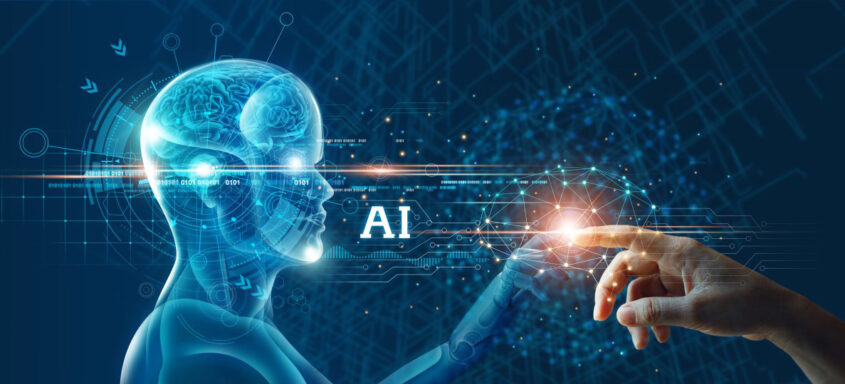
Table of Contents
AI is not truly intelligent, it's sophisticated pattern recognition.
The myth of general intelligence
Current AI systems lack general intelligence; they operate within narrow domains. They excel at specific tasks but struggle with others requiring adaptability or common sense. This crucial distinction highlights the difference between narrow (weak) AI and general (strong) AI.
- AI excels at: Image recognition, natural language processing (NLP) for specific tasks like translation, playing games like chess or Go (often surpassing human capabilities within those very specific rulesets), and specific automation tasks.
- AI struggles with: Understanding context in complex situations, adapting to unforeseen circumstances, exhibiting common sense reasoning, and transferring knowledge from one domain to another.
The term "Artificial General Intelligence" (AGI) refers to a hypothetical AI with human-level cognitive abilities. We are far from achieving AGI. Current AI is essentially advanced pattern recognition – identifying and extrapolating patterns from massive datasets.
AI relies on vast datasets and complex algorithms
The apparent intelligence of AI stems from processing massive amounts of data and sophisticated algorithms, not from genuine understanding or consciousness. The algorithms identify correlations and probabilities within the data, allowing them to make predictions and perform tasks seemingly intelligently.
- Data requirements: Training a powerful image recognition model requires millions of labeled images. Similarly, advanced NLP models are trained on billions of words of text.
- Limitations of data-driven approaches: AI models are vulnerable to biases present in the training data, leading to unfair or inaccurate outcomes. They also often lack the ability to understand context or apply knowledge outside the specific data they were trained on. This limitation results in brittle systems that can fail spectacularly when confronted with situations outside their training parameters.
The more data and the more sophisticated the algorithm, the more impressive the performance can appear; however, it doesn't equate to genuine intelligence.
AI lacks common sense and real-world understanding.
The challenge of context and ambiguity
AI struggles significantly with nuanced situations requiring common sense or understanding of context. Human intelligence easily handles ambiguity and incorporates background knowledge, but this is a major challenge for current AI systems.
- Examples of AI failures: A self-driving car might fail to navigate an unusual road obstruction or misinterpret a seemingly simple traffic sign because it hasn't encountered that specific scenario in its training data. A chatbot might respond inappropriately to a nuanced or sarcastic question, lacking the contextual understanding necessary for a suitable reply.
- Programming common sense: Encoding common sense into AI systems is extremely difficult. It involves representing a vast body of implicit knowledge and understanding of the world, a task far beyond current AI capabilities.
AI's inability to reason and understand causality
AI often struggles with tasks requiring complex reasoning, causal inference, and understanding the implications of actions. While it can process information and identify correlations, it doesn't inherently understand causality or the "why" behind events.
- Examples: An AI might predict that event A is likely to precede event B based on statistical correlations, but it won't necessarily understand the underlying causal relationship between them. It might be able to identify patterns that indicate a medical condition, but it can't diagnose or treat the condition itself without explicit programming by experts.
- Ongoing research: Research into causal inference and reasoning is ongoing and crucial for advancing the field. However, achieving human-like levels of causal understanding remains a significant challenge.
AI is not autonomous or self-aware.
The role of human input and oversight
AI systems are tools developed and controlled by humans. Their actions are ultimately determined by human design, data, and programming. Although AI can automate certain tasks, it's not truly autonomous, requiring significant human input and oversight.
- Examples of human intervention: AI systems often require human feedback during the training process, human intervention to correct errors, and ongoing human monitoring to ensure ethical and safe operation.
- Ethical considerations: The increasing autonomy of AI systems raises ethical considerations regarding accountability, bias, and the potential for unintended consequences. The lack of true autonomy and the vital role of human oversight are key to mitigating these risks.
The absence of consciousness and subjective experience
Current AI lacks consciousness, self-awareness, and subjective experience. It operates based on algorithms and data, not on feelings, emotions, or self-reflection. This fundamental difference separates AI from human intelligence.
- AI vs. Human Consciousness: AI can mimic human-like conversation or creative output, but it doesn't possess the internal subjective experience or consciousness that underpins human intelligence.
- Philosophical debate: The question of whether AI can ever achieve consciousness remains a topic of philosophical debate, but current systems are demonstrably far from such a state.
Conclusion
This article has highlighted the key aspects of the "Illusion of AI Intelligence." We've explored AI's reliance on pattern recognition, its lack of common sense and general intelligence, and its dependence on human input. The remarkable achievements of AI should be celebrated, but we must avoid overestimating its capabilities. Let's move beyond the illusion of AI intelligence and foster a more realistic and responsible approach to AI development and deployment. A clearer understanding of AI's limitations is crucial for its ethical and beneficial integration into society.
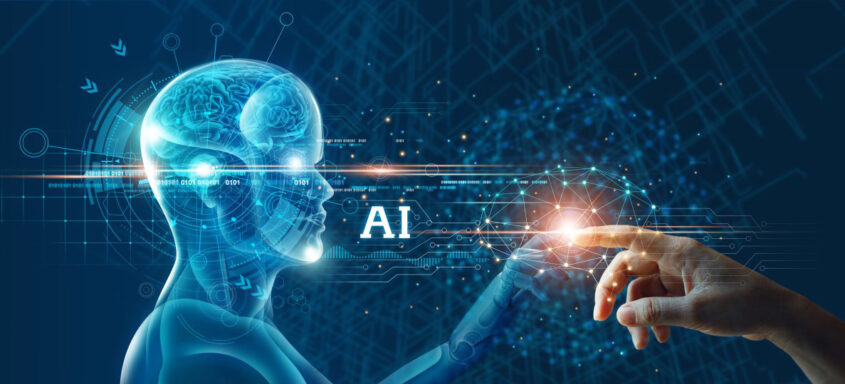
Featured Posts
-
Filipino Community In Canada Reeling From Car Ramming Tragedy
Apr 29, 2025 -
Search Underway For Missing British Paralympian Sam Ruddock In Las Vegas
Apr 29, 2025 -
911 Cayenne
Apr 29, 2025 -
Soaring Costs Prompt Lynas Rare Earths To Seek Us Funding For Texas Plant
Apr 29, 2025 -
Post Trump Cuts A Global Fight For American Scientific Talent
Apr 29, 2025
Latest Posts
-
Klagenfurt Im Sturzflug Die Aktuelle Lage In Der Bundesliga
Apr 29, 2025 -
Qualifikationsgruppe Gewonnen Lask Feiert 6 0 Erfolg Gegen Klagenfurt
Apr 29, 2025 -
Klagenfurts Abstiegskampf Investor Plant Trainertausch Jancker Im Fokus
Apr 29, 2025 -
Champions League Kaiserslautern Und Die Herausforderung Bayern Muenchen
Apr 29, 2025 -
Klarer Sieg Fuer Lask 6 0 Gegen Klagenfurt Bedeutet Gruppensieg
Apr 29, 2025