The Impact Of Outdated Business Applications On AI Initiatives
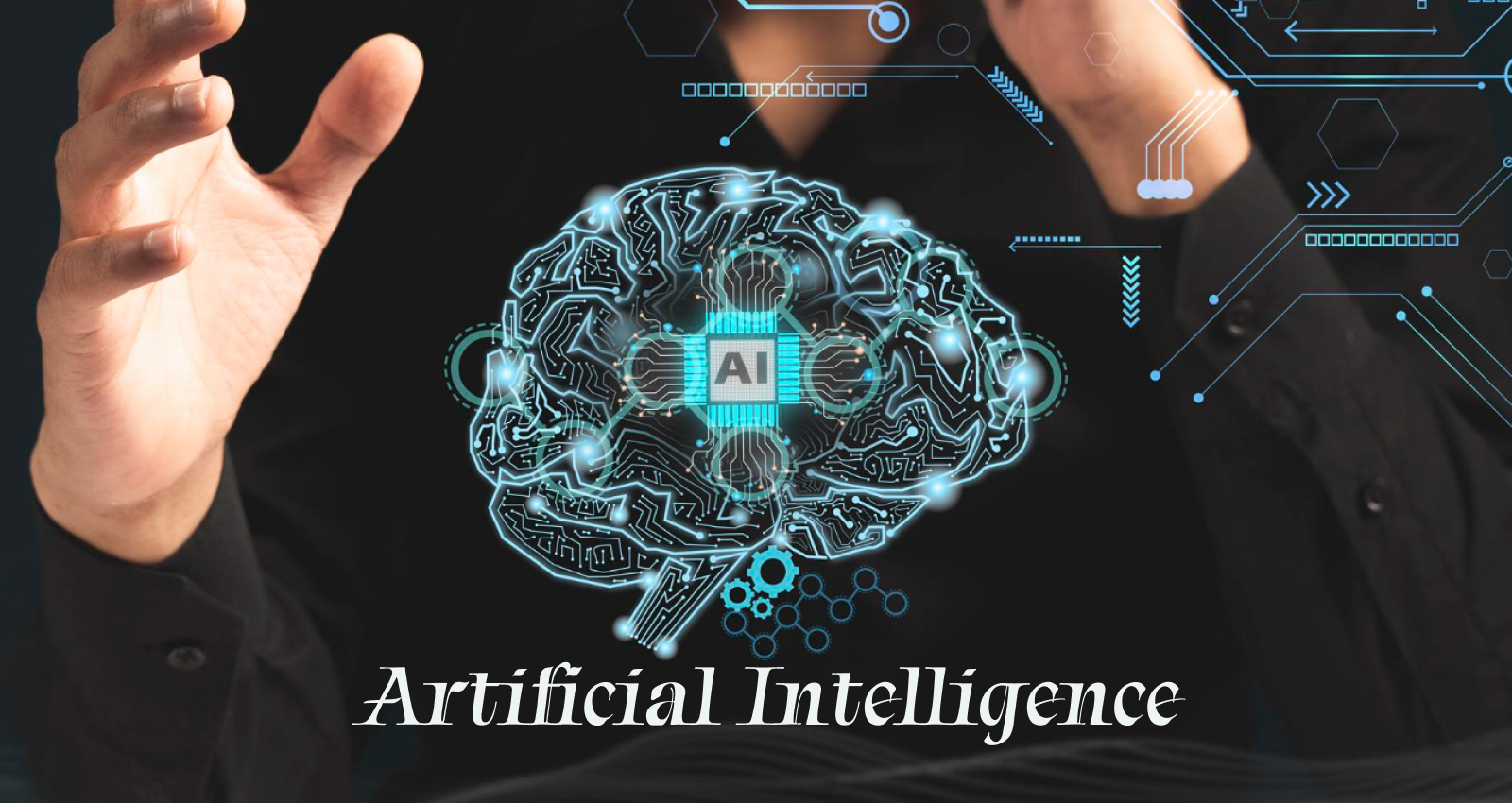
Table of Contents
Data Silos and Incompatibility: A Major Hurdle for AI
Legacy systems often create significant data silos, hindering the seamless flow of information necessary for effective AI implementation. These systems frequently lack the interoperability needed to integrate data from various sources, creating a fragmented data landscape unsuitable for training robust AI models. The incompatibility between old systems and modern AI tools further exacerbates this problem.
- Lack of standardized data formats: Inconsistencies in data formats across different systems require extensive data cleaning and transformation, significantly increasing AI project timelines and costs.
- Difficulty in data extraction and migration: Extracting data from legacy systems is often a complex and time-consuming process, requiring specialized expertise and potentially disrupting ongoing operations.
- Increased complexity in data cleaning and preprocessing: The poor quality and inconsistent nature of data from legacy systems demand significant effort in cleaning and preprocessing before it can be used for AI training.
- Example: Imagine an outdated CRM system failing to integrate with a new AI-powered marketing automation platform. This prevents the AI from leveraging valuable customer data for personalized marketing campaigns, limiting its effectiveness.
Scalability and Performance Limitations of Legacy Systems
Outdated systems often struggle to handle the vast datasets required for effective AI training and deployment. The sheer volume of data needed to train sophisticated AI models often exceeds the processing power and storage capacity of legacy infrastructure. This results in significant performance bottlenecks that can severely impact AI project success.
- Slow processing speeds impacting AI model training time: Training complex AI models can take days, weeks, or even months on underpowered legacy systems, significantly delaying project completion.
- Limited storage capacity hindering data storage and retrieval: Legacy systems may lack the storage capacity to accommodate the large datasets needed for effective AI model training and deployment.
- Increased risk of system failures during high-volume AI operations: The strain of processing large datasets on outdated infrastructure can lead to system failures and data loss, disrupting AI operations.
- Example: A legacy ERP system might be unable to cope with the increased data volume generated by an AI-powered supply chain optimization tool, leading to inaccurate predictions and missed opportunities.
Security Risks and Compliance Issues in Outdated Applications
Legacy systems often present significant security vulnerabilities, making them susceptible to breaches and data loss. This is particularly critical for AI initiatives involving sensitive customer or employee data. Furthermore, many outdated systems fail to comply with modern data privacy regulations, exposing organizations to significant legal and financial risks.
- Outdated security protocols increasing vulnerability to cyberattacks: Legacy systems frequently lack up-to-date security protocols, making them easy targets for cybercriminals.
- Lack of data encryption leading to data breaches: The absence of robust data encryption in legacy systems increases the risk of data breaches and exposes sensitive information to unauthorized access.
- Non-compliance with data privacy regulations leading to penalties: Outdated systems may not comply with regulations like GDPR or CCPA, resulting in hefty fines and reputational damage.
- Example: A legacy HR system storing sensitive employee data without sufficient encryption could hinder the use of AI in HR analytics due to data privacy concerns.
The High Cost of Maintaining Outdated Systems and its Impact on AI Investment
Maintaining legacy systems involves substantial hidden costs, including ongoing maintenance, upgrades, and the lack of vendor support. These expenses often divert valuable resources that could be allocated to crucial AI initiatives. The cost of inaction significantly outweighs the cost of modernization.
- High maintenance costs diverting funds from AI projects: The ongoing costs of maintaining legacy systems can drain budgets, leaving less funding available for AI development and deployment.
- Lack of vendor support hindering system upgrades: Many legacy systems lack vendor support, making upgrades and security patches difficult or impossible to implement.
- Increased downtime and reduced productivity: Outdated systems are more prone to downtime and malfunctions, leading to reduced productivity and lost revenue.
- Example: An organization might spend a significant budget on maintaining an outdated database system instead of investing in a cloud-based AI solution that offers superior scalability, performance, and security.
Strategies for Modernization: Preparing Your Business for Successful AI Integration
Modernizing your IT infrastructure is essential for successful AI integration. This involves migrating to modern applications that support AI's data-intensive nature and complex processing requirements.
- Cloud migration for improved scalability and performance: Migrating to the cloud provides the scalability and performance needed to handle large datasets and complex AI models.
- Implementation of data integration tools for seamless data flow: Data integration tools can connect disparate data sources, eliminating data silos and facilitating the creation of a unified data lake for AI training.
- Utilizing APIs to connect legacy systems with modern AI platforms: APIs can be used to bridge the gap between legacy systems and modern AI platforms, allowing for gradual modernization without a complete system overhaul.
- Phased approach to system modernization to minimize disruption: A phased approach to system modernization allows organizations to migrate to modern systems incrementally, minimizing disruptions to ongoing operations.
Conclusion: Overcoming the Obstacles: A Roadmap to AI Success
Outdated business applications present significant hurdles to successful AI implementation. Data silos, scalability limitations, security risks, and high maintenance costs can severely impede AI initiatives. Modernizing your IT infrastructure is not just an option; it's a necessity for harnessing the transformative power of AI. By addressing the impact of outdated business applications on AI initiatives, organizations can unlock the full potential of AI and gain a competitive edge. Ready to modernize for AI success? Assess your current infrastructure and begin planning your modernization strategy today. [Link to relevant resource/consultation]
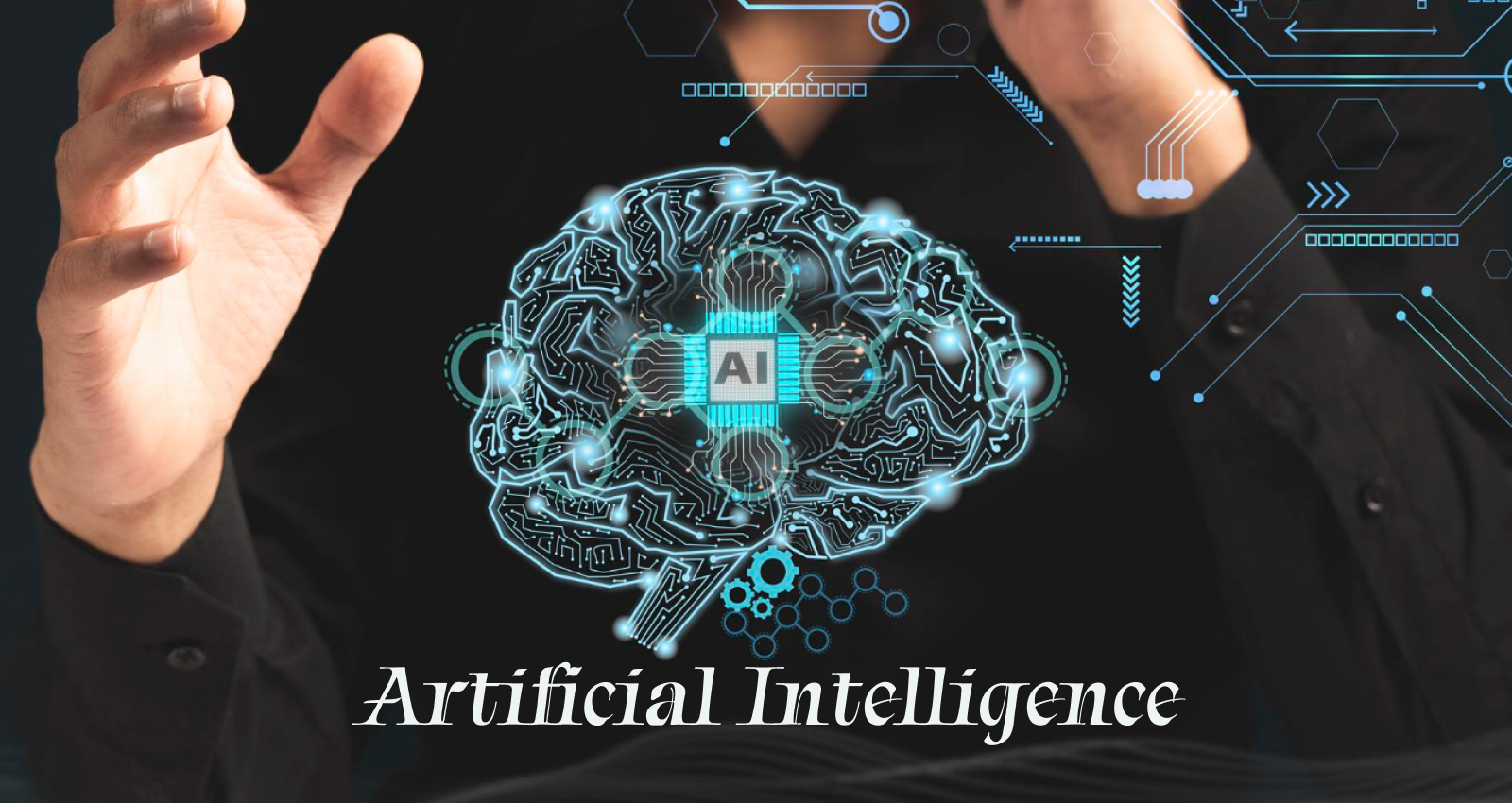
Featured Posts
-
Ripples Xrp Navigating The Sec Case And The Potential Of Etfs
May 01, 2025 -
Michael Jordan Fast Facts And Key Stats
May 01, 2025 -
Ex Wallaby Claims Australian Rugby Lacks Hemispheric Dominance
May 01, 2025 -
Priscilla Pointer Amy Irvings Mother And Actress In Carrie Dies At 100
May 01, 2025 -
Noa Argamanis Journey From Hostage To Time 100 Influencer
May 01, 2025
Latest Posts
-
Free Speech Debate Police Officers Tweet On Chris Rock Sparks Investigation
May 01, 2025 -
Black And Asian Police Leader Faces Investigation Following Tweet About Chris Rock
May 01, 2025 -
Ultima Oportunidad Clases De Boxeo En Edomex 3 Dias
May 01, 2025 -
Police Leader Investigated Over Chris Rock Tweet Free Speech Concerns
May 01, 2025 -
Clases De Boxeo En Edomex Inscripcion En 3 Dias
May 01, 2025