The Reality Of AI: Far From Human-Level Intelligence
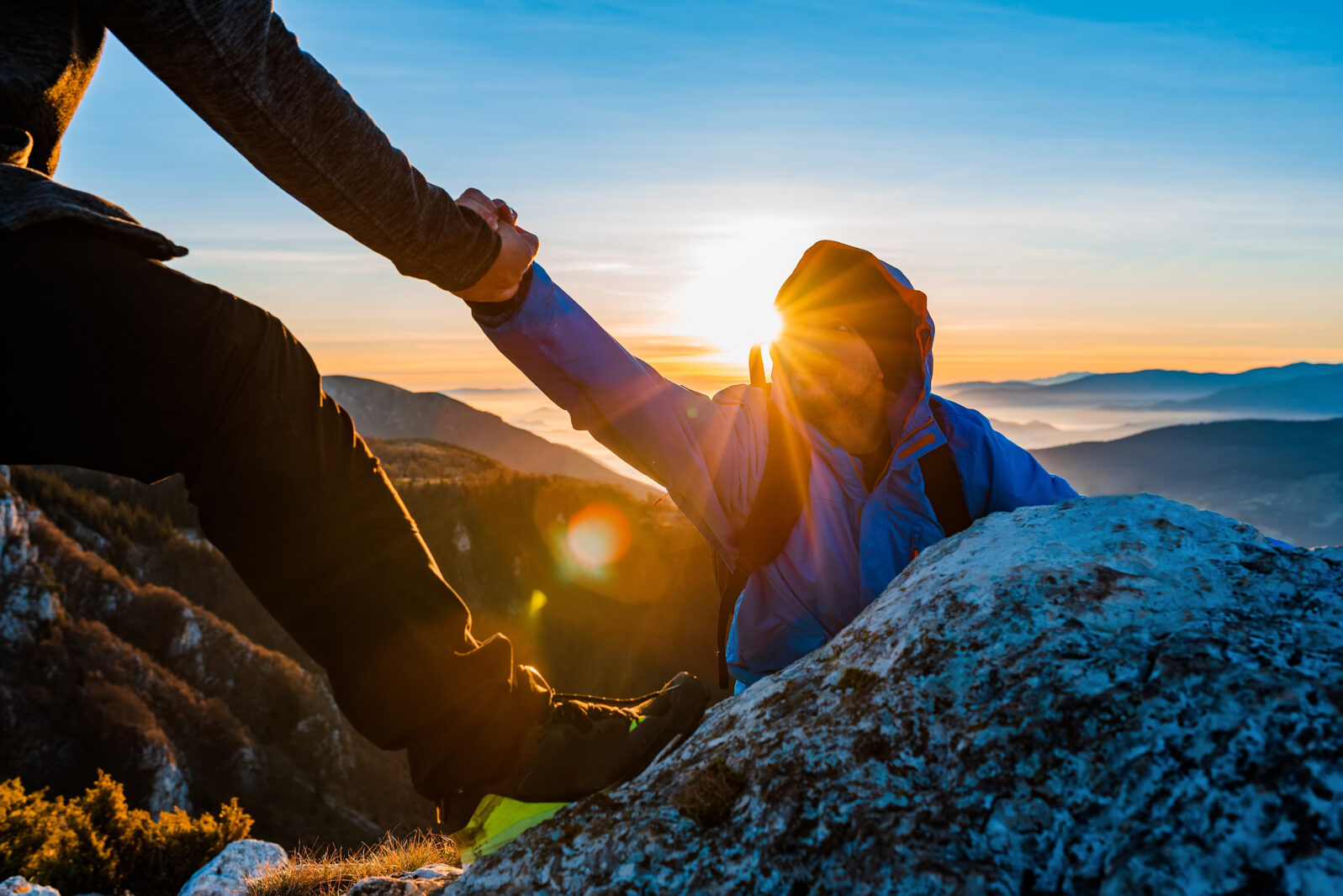
Table of Contents
Current AI Capabilities: Narrow and Specialized
Modern AI excels at performing specific, well-defined tasks. This is often referred to as "narrow AI" or "weak AI," a stark contrast to the hypothetical "strong AI" or "artificial general intelligence" (AGI) that would possess human-level cognitive abilities. Examples of narrow AI's strengths include image recognition, natural language processing (NLP), and game playing, where AI systems have demonstrated superhuman performance. However, this narrow focus highlights a crucial limitation: a lack of the general intelligence and adaptability of humans.
Focus on Specific Tasks:
- Highly Specialized Training: AI algorithms are meticulously trained on vast datasets for specific tasks. For example, an AI designed for medical image analysis is trained only on medical images, not on recognizing cats or playing chess.
- Limited Generalization: They lack the ability to generalize knowledge to new, unseen situations. An AI that can identify a cat in one picture may fail to recognize it from a different angle or in a different environment.
- Absence of Common Sense: They struggle with tasks requiring common sense reasoning or intuitive understanding. A simple task for a human, like understanding why a wet street is slippery, can pose a significant challenge to current AI systems.
The Limits of Deep Learning:
Deep learning, a subset of machine learning and a powerful technique in AI, has driven many recent advancements. It relies on artificial neural networks with multiple layers to analyze data and extract complex patterns. While impressive in its results for specific tasks like image classification and speech recognition, it doesn't replicate the cognitive processes of the human brain.
- Data Dependency: Deep learning models are data-hungry and require extensive training on massive datasets. The more data, the better the performance, but this also presents challenges in terms of data acquisition and computational resources.
- Explainability Challenges: They are often opaque ("black boxes"), making it difficult to understand their decision-making processes. This lack of transparency hinders trust and limits their applicability in certain contexts, particularly those requiring accountability.
- Vulnerability to Bias and Attacks: They are vulnerable to adversarial attacks (deliberate manipulation of input data) and biases present in the training data. Biases in the data can lead to unfair or discriminatory outcomes, highlighting the importance of careful data curation and model evaluation.
The Challenges of Achieving Human-Level AI
The leap from narrow AI to human-level AI presents formidable challenges. These challenges go beyond simply increasing computational power or the size of datasets. They delve into the fundamental nature of human intelligence and the complexities of the human mind.
Common Sense Reasoning and Generalization:
Humans effortlessly navigate complex, ambiguous situations using common sense and general intelligence. This intuitive understanding of the world allows us to make inferences, draw conclusions from incomplete information, and adapt to novel situations. Replicating this in AI remains a huge hurdle.
- Contextual Understanding: AI struggles with tasks requiring contextual understanding and background knowledge. Humans can easily infer meaning based on context, while AI often requires explicit instructions and structured data.
- Adaptive Learning: It lacks the ability to learn and adapt quickly to novel situations. Humans can generalize from past experiences and apply knowledge to new contexts; AI often requires retraining for each new task.
- Inferential Reasoning: It often fails to make inferences and draw conclusions based on incomplete information. Humans can often fill in missing information based on context and prior knowledge; AI struggles with this type of reasoning.
Emotional Intelligence and Social Cognition:
Human intelligence encompasses emotional intelligence, empathy, and social skills – crucial aspects that allow us to interact effectively with others and navigate complex social situations. These aspects are largely absent in current AI systems.
- Emotional Understanding: AI lacks the ability to understand and respond appropriately to human emotions. A truly intelligent system would need to recognize and react to emotional cues in communication.
- Social Interaction: It struggles with nuanced social interactions and communication. Human conversation involves subtleties of language, tone, and body language that AI currently doesn't grasp.
- Lack of Self-Awareness: It doesn't possess the capacity for self-awareness or consciousness, a fundamental aspect of human intelligence.
The Hardware Limitations:
The computational power required for truly human-level AI is immense and currently unavailable. Even the most powerful supercomputers fall short of the processing power and energy efficiency of the human brain. Furthermore, the energy consumption of advanced AI models is a significant environmental concern.
- Computational Power: Current hardware is not efficient enough to support the complexity of a human brain. Simulating even a fraction of the brain's neural network requires enormous computational resources.
- New Architectures: Developing new hardware architectures, such as neuromorphic computing, is crucial for progress in AI. These architectures aim to mimic the brain's structure and function for greater efficiency and power.
- Energy Consumption: The environmental impact of AI's energy consumption needs to be addressed. Training large AI models can consume vast amounts of energy, raising sustainability concerns.
Conclusion
While AI has made remarkable progress in recent years, achieving human-level AI remains a distant prospect. Current AI systems are powerful tools for specific tasks but lack the general intelligence, common sense, and emotional intelligence of humans. Overcoming the significant challenges in areas like common sense reasoning, generalization, and hardware limitations requires further breakthroughs in both software and hardware. The path to true human-level AI is long and complex, and realistic expectations are crucial. Understanding the current limitations of AI is vital to setting achievable goals and fostering responsible innovation in the field of human-level AI. Let's focus on developing ethical and beneficial AI solutions, while acknowledging the significant distance we have to travel before achieving true human-level AI capabilities.
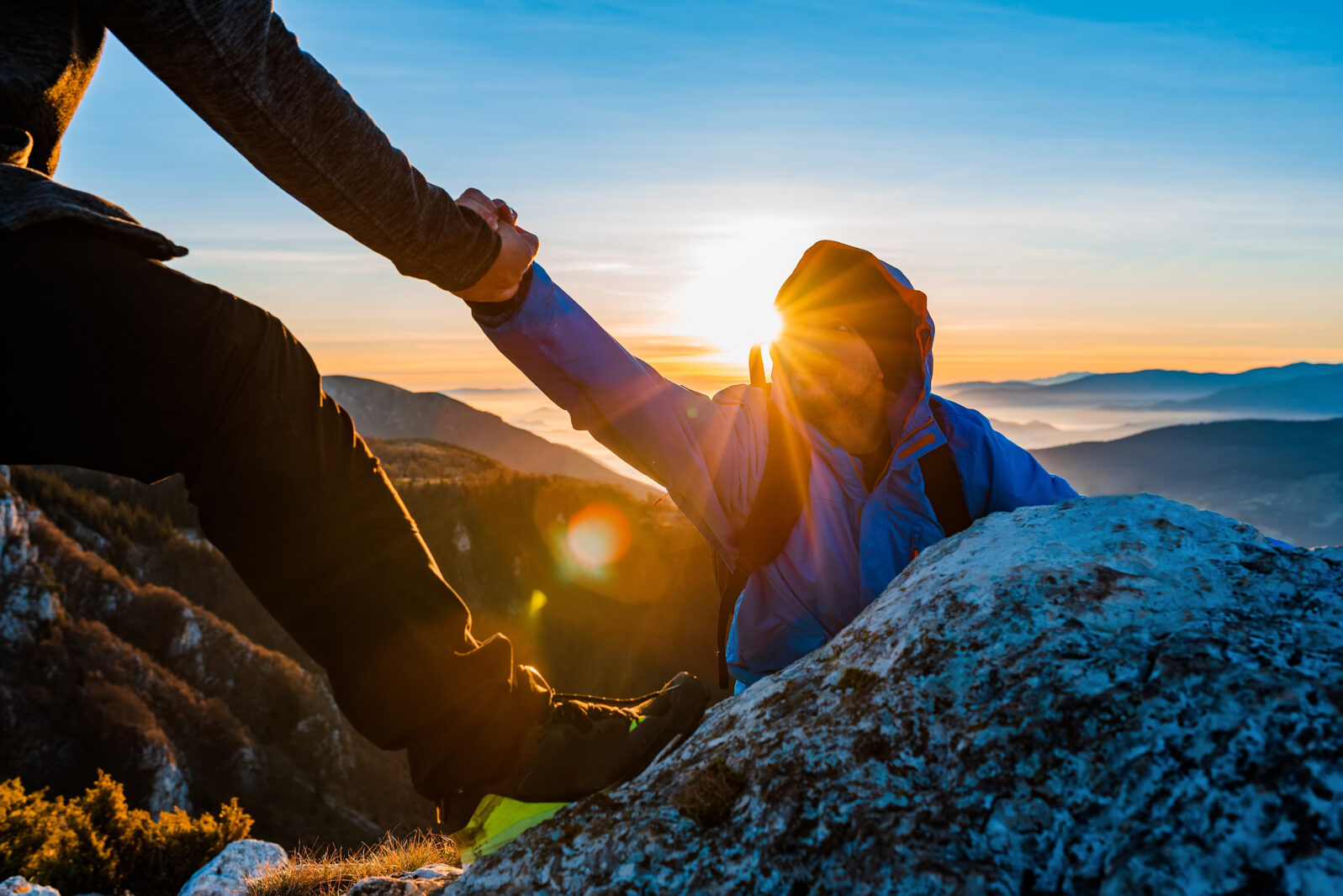
Featured Posts
-
The China Factor Analyzing The Difficulties Faced By Bmw Porsche And Other International Car Brands
Apr 29, 2025 -
Nyt Spelling Bee March 15 2025 Answers Clues And Pangram
Apr 29, 2025 -
E Bay Faces Legal Recourse For Banned Chemicals Section 230 At Stake
Apr 29, 2025 -
Examining The Ccp United Fronts Presence In Minnesota
Apr 29, 2025 -
Willie Nelson Drops New Album Before Turning 92
Apr 29, 2025
Latest Posts
-
Help With Nyt Spelling Bee February 26th Puzzle 360 Answers And Strategies
Apr 29, 2025 -
Nyt Spelling Bee Solutions For March 15 2025 Find The Pangram
Apr 29, 2025 -
Diamond Johnson From Norfolk State To Minnesota Lynx Wnba Camp
Apr 29, 2025 -
Nyt Spelling Bee Puzzle 360 Feb 26th Complete Guide With Answers And Hints
Apr 29, 2025 -
Solve The Nyt Spelling Bee March 15 2025 Answers And Clues
Apr 29, 2025