Understanding AI Cognition: A Look At The Current Limitations
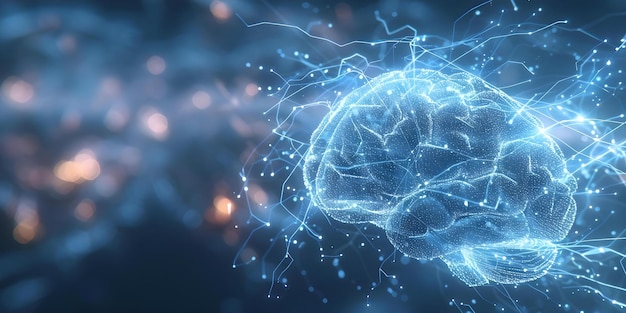
Table of Contents
The Lack of Common Sense Reasoning in AI
AI systems, while powerful in specific domains, often struggle with tasks requiring common sense reasoning. This fundamental gap highlights crucial limitations in AI cognition.
Limited Contextual Understanding
AI struggles with nuanced situations demanding common sense reasoning. It often fails to grasp implicit information or make inferences based on everyday knowledge. For instance, an AI might struggle to understand why leaving a wet umbrella inside is a bad idea, lacking the common sense understanding of water damage. This lack of contextual understanding stems from limitations in knowledge representation and the difficulty of encoding common sense knowledge into AI systems. Effective AI cognition requires a richer understanding of the world beyond explicitly programmed facts.
- Keyword integration: Common sense reasoning, AI limitations, contextual understanding, knowledge representation.
- Key challenges: Encoding implicit knowledge, handling ambiguity, understanding unspoken assumptions.
Difficulty with Abstract Concepts
AI systems typically excel at specific tasks but find abstract concepts challenging. Understanding metaphors, humor, or sarcasm often proves difficult. For example, an AI might misinterpret a sarcastic remark as a literal statement, demonstrating a lack of nuanced understanding of human communication. This limitation highlights the challenges in enabling AI to process and understand symbolic reasoning and the complexities of human language.
- Keyword integration: Abstract reasoning, AI challenges, symbolic reasoning, semantic understanding.
- Key challenges: Interpreting figurative language, understanding implied meaning, processing complex narratives.
Brittleness in Unseen Scenarios
AI models are often trained on specific datasets and perform poorly when presented with situations outside their training data. A self-driving car trained on sunny weather conditions might struggle to navigate in heavy rain or snow, highlighting the brittleness of many current AI systems. This lack of robustness and generalization is a significant hurdle in developing truly intelligent AI. Improving the generalizability of AI models is a key area of ongoing research.
- Keyword integration: Robustness, generalization, AI vulnerability, out-of-distribution data.
- Key challenges: Developing more robust algorithms, creating more diverse training datasets, improving model adaptation capabilities.
The Absence of True Consciousness and Self-Awareness
A crucial aspect of human intelligence, often overlooked in the discussion of AI cognition, is consciousness and self-awareness. Current AI systems demonstrably lack these qualities.
The Hard Problem of Consciousness
AI lacks subjective experience and sentience. While AI can mimic human behavior, it does not possess the same internal feelings or self-awareness. An AI can generate human-like text, but it does not understand the meaning or emotional context behind its own words. This "hard problem of consciousness" remains a significant philosophical and scientific challenge.
- Keyword integration: Consciousness, sentience, subjective experience, AI philosophy.
- Key challenges: Defining consciousness scientifically, developing methods to measure consciousness in AI, understanding the relationship between brain structure and consciousness.
The Limits of Emotional Intelligence
Although some progress is being made in emotion recognition, AI lacks genuine emotional understanding and empathy. An AI chatbot can identify sadness in text, but it cannot truly feel or respond to that sadness with empathy. This absence of emotional intelligence limits the ability of AI to interact meaningfully with humans in complex social situations.
- Keyword integration: Emotional intelligence, empathy, affective computing, human-computer interaction.
- Key challenges: Developing AI systems capable of understanding and responding to emotions appropriately, creating AI with the ability to empathize with human experiences.
Ethical Considerations and Biases in AI Cognition
The development and deployment of AI systems raise significant ethical considerations, particularly concerning bias and transparency.
Bias in Training Data
AI systems inherit the biases present in the data they are trained on, leading to unfair or discriminatory outcomes. Facial recognition systems trained on predominantly white faces may perform poorly on people with darker skin tones, illustrating the dangers of biased datasets. Addressing this bias is crucial for ensuring fairness and equity in AI applications.
- Keyword integration: AI ethics, bias mitigation, fairness, algorithmic bias.
- Key challenges: Identifying and mitigating biases in training data, developing algorithms that are less susceptible to bias, ensuring fairness and accountability in AI systems.
Lack of Transparency and Explainability
The decision-making processes of complex AI models are often opaque, making it difficult to understand why they reach particular conclusions. A loan application might be rejected by an AI system without a clear explanation for the decision, raising concerns about accountability and fairness. Developing explainable AI (XAI) is crucial for building trust and ensuring responsible AI development.
- Keyword integration: Explainable AI (XAI), transparency, interpretability, accountability.
- Key challenges: Developing methods to make AI decision-making processes more transparent, creating models that provide clear and understandable explanations for their outputs, balancing transparency with the need for model security.
Conclusion
Understanding AI cognition highlights the significant limitations of current AI systems. While AI has made remarkable strides, it still lacks crucial aspects of human intelligence, such as common sense reasoning, consciousness, and emotional intelligence. Furthermore, ethical concerns surrounding bias and transparency must be addressed. Continued research and development are crucial to overcome these limitations and build more robust, ethical, and truly intelligent AI systems. To stay informed about the advancements and challenges in this rapidly evolving field, continue exploring the intricacies of AI cognition and its potential future. Understanding the limitations of AI cognitive abilities is paramount for responsible innovation.
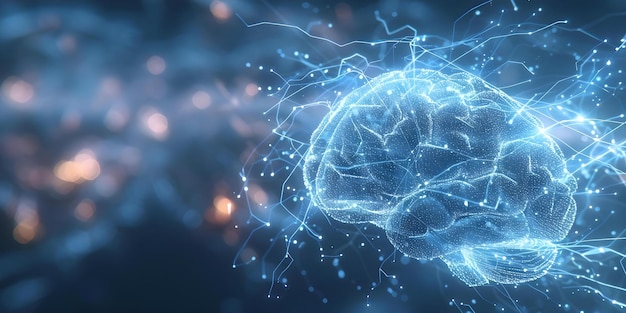
Featured Posts
-
Betting On Natural Disasters The Los Angeles Wildfires And The Changing Landscape Of Gambling
Apr 29, 2025 -
Blue Origin Scraps Rocket Launch Due To Subsystem Problem
Apr 29, 2025 -
Nyt Spelling Bee March 14 2025 Solutions Answers And Pangram
Apr 29, 2025 -
Nyt Strands Hints And Answers March 3 2025
Apr 29, 2025 -
Conquer Nyt Strands February 27 2025 Hints And Answers
Apr 29, 2025
Latest Posts
-
Nyt Spelling Bee April 3 2025 Complete Answers And Pangram Reveal
Apr 29, 2025 -
Nyt Strands Today April 1 2025 Clues And Solutions
Apr 29, 2025 -
Nyt Strands Hints And Answers March 3 2025
Apr 29, 2025 -
March 3 2025 Nyt Strands Finding The Solutions
Apr 29, 2025 -
Solve The Nyt Spelling Bee April 3 2025 Answers And Strategies
Apr 29, 2025