We Now Know How AI "Thinks"—and It's Barely Thinking At All
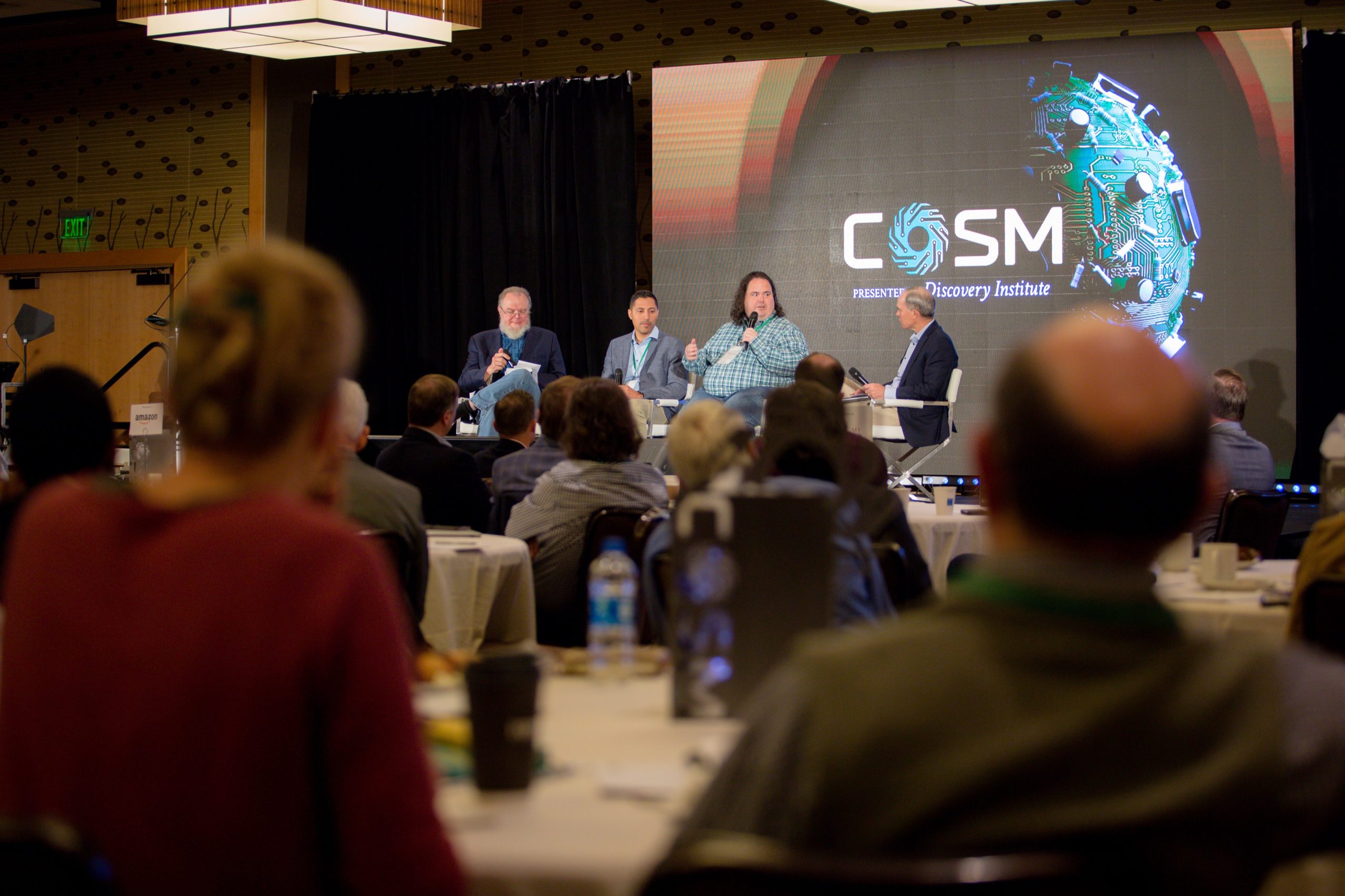
Table of Contents
Keywords: AI thinking, artificial intelligence, machine learning, deep learning, AI limitations, AI capabilities, AI consciousness, narrow AI, general AI, AI algorithms.
The term "artificial intelligence" evokes images of sentient machines, capable of independent thought and complex reasoning. Sci-fi movies fuel this perception, portraying AI as conscious entities with human-like intelligence. But the reality of how AI "thinks" is far more nuanced and, frankly, less intelligent than many believe. This article delves into the current state of AI, revealing its surprising limitations and exposing the gap between popular perception and actual capabilities. We'll explore the mechanisms behind AI's apparent intelligence, its current shortcomings, and the exciting – and ethically complex – path toward a truly intelligent future.
The Illusion of Intelligence: How AI Works
Statistical Pattern Recognition, Not True Understanding
AI systems, at their core, operate by identifying patterns in vast datasets. This is achieved through sophisticated algorithms, but it's crucial to understand that this pattern recognition is fundamentally different from human understanding. AI doesn't "understand" the data in the human sense; it identifies statistical correlations.
-
Statistical methods: Machine learning relies heavily on statistical methods like:
- Regression: Predicting a continuous value (e.g., house price prediction).
- Classification: Assigning data points to categories (e.g., spam detection).
- Clustering: Grouping similar data points together (e.g., customer segmentation).
-
Example: Image Recognition: An AI system might identify a cat in an image by recognizing recurring pixel patterns associated with cats in its training data. It doesn't inherently "know" what a cat is; it simply recognizes patterns that correlate with the label "cat." This is a critical distinction in understanding AI thinking. The system is not capable of abstract thought or conceptual understanding.
The Role of Training Data and Bias
AI's performance is profoundly dependent on the quality and representativeness of its training data. Garbage in, garbage out, as the saying goes. Furthermore, biases present in the training data will inevitably lead to biased outputs. This is a significant limitation and a major area of concern in the field.
-
Biased Data, Biased Outcomes: If a facial recognition system is trained primarily on images of one demographic group, it will likely perform poorly when recognizing individuals from underrepresented groups. This is a real-world example of how biased training data leads to unfair and inaccurate results.
-
Data Scarcity and Representation: The lack of diverse and representative datasets is a major obstacle to developing fair and reliable AI systems. Addressing this requires careful data collection and curation, ensuring inclusivity and mitigating potential biases. The impact of poor representation extends across many AI applications, from loan applications to medical diagnoses.
Limitations of Current AI Systems
Narrow vs. General AI
A key distinction to understand is the difference between narrow AI and general AI.
-
Narrow AI: Current AI systems are almost exclusively narrow AI, meaning they are designed and trained to perform a specific task or a narrow set of tasks. They excel within their limited domain but lack the adaptability and general intelligence of humans. Think of a chess-playing AI – it can beat grandmasters, but it can't drive a car or write a poem.
-
General AI: General AI (AGI), also known as artificial general intelligence, is a hypothetical AI with human-level intelligence. It would possess the ability to learn, reason, and solve problems across a wide range of domains, just like a human. AGI remains a distant goal, with significant technological and conceptual hurdles to overcome.
Lack of Common Sense and Reasoning
Current AI systems often struggle with tasks that seem trivial to humans, such as common sense reasoning and real-world problem-solving. They lack the intuitive understanding of the world that comes naturally to humans.
-
Challenges with Analogy and Context: AI systems frequently fail to grasp analogies or interpret contextual information correctly. For example, an AI might struggle to understand the meaning of a sentence if it's slightly out of the ordinary or uses figurative language.
-
The Difficulty of Programming Common Sense: The challenge of encoding common sense into AI systems is one of the most significant obstacles in the pursuit of general AI. Common sense is not easily codified; it's a complex interplay of experience, knowledge, and intuition.
The Future of AI Thinking
The Pursuit of General AI
The development of general AI remains a long-term goal, driving much of the ongoing research in artificial intelligence.
-
Key Research Areas: Researchers are exploring various avenues to achieve AGI, including:
- Advanced Neural Networks: Developing more sophisticated and powerful neural network architectures.
- Cognitive Architectures: Creating models that mimic the structure and function of the human brain.
- Symbolic AI: Combining statistical methods with symbolic reasoning techniques.
-
Ethical Implications: The creation of truly intelligent AI raises profound ethical questions, requiring careful consideration of potential risks and benefits.
Responsible Development and Deployment of AI
The responsible development and deployment of AI are paramount.
-
Addressing Bias and Ensuring Transparency: It is crucial to mitigate biases in AI systems and ensure transparency in their decision-making processes. This requires ongoing monitoring, evaluation, and refinement of AI algorithms and datasets.
-
The Role of Regulation and Responsible AI Practices: Regulations and ethical guidelines are necessary to ensure that AI is developed and used responsibly, promoting fairness, accountability, and societal well-being.
-
Societal Impact: The widespread adoption of AI will have profound implications for society, impacting employment, healthcare, education, and many other aspects of life. Careful planning and proactive measures are needed to navigate these changes effectively.
Conclusion
This article has explored the fascinating yet limited nature of current AI "thinking." While AI systems can achieve remarkable feats through pattern recognition, they lack true understanding, common sense, and general intelligence. They excel at specific tasks but fall short when faced with the complexities and nuances of the real world.
Call to Action: Understanding how AI actually "thinks" – and its inherent limitations – is crucial for responsible development and deployment. Continue learning about the advancements and challenges in artificial intelligence and contribute to the ethical discussion surrounding its future. Learn more about the complexities of AI thinking and its impact on our world, and help shape a future where AI benefits all of humanity.
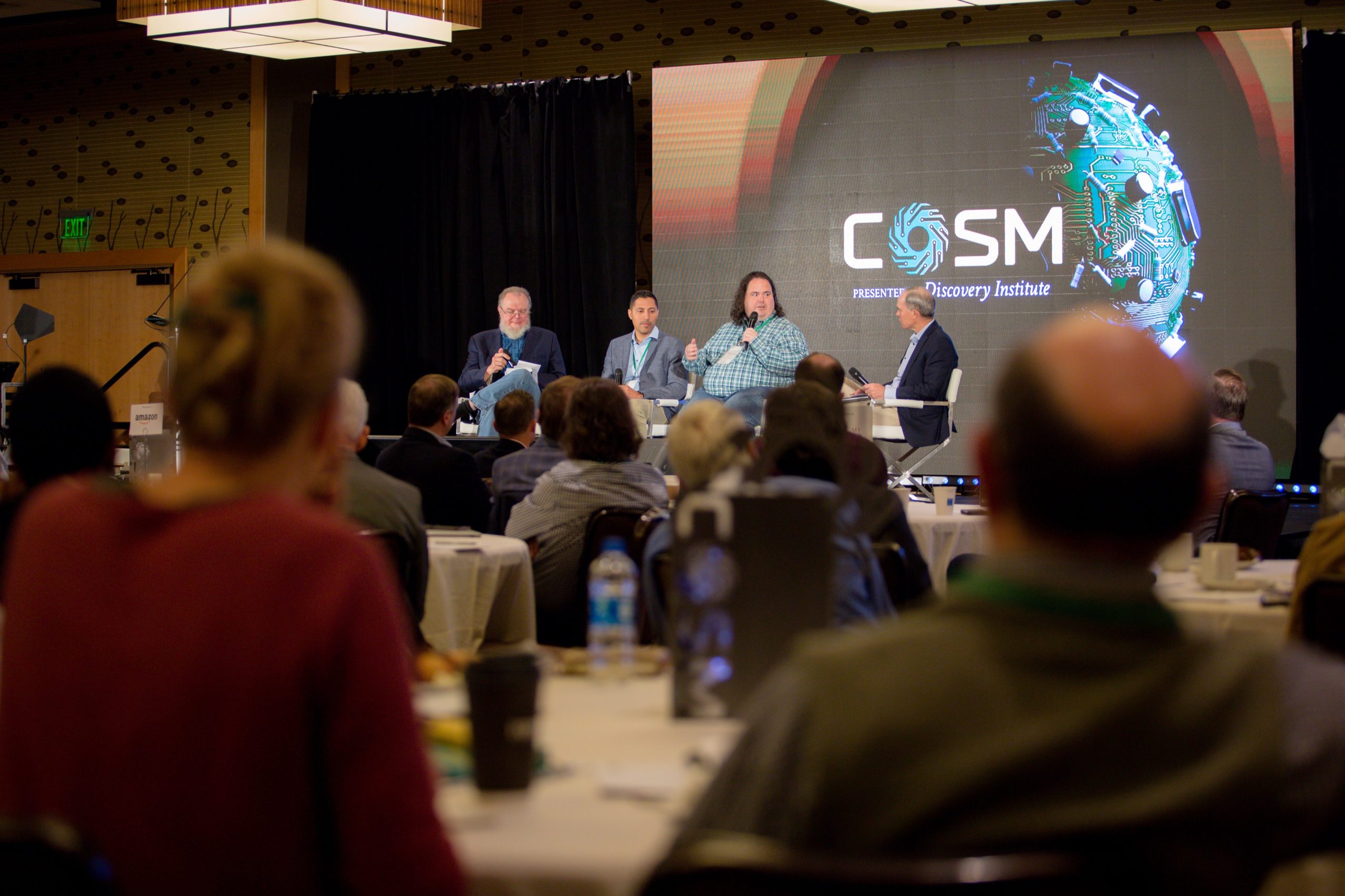
Featured Posts
-
Trumps Transgender Athlete Ban Us Attorney General Targets Minnesota
Apr 29, 2025 -
San Franciscos Anchor Brewing Company Announces Closure
Apr 29, 2025 -
Speedboat Flips During Arizona Boating Competition Record Attempt Video
Apr 29, 2025 -
New Business Hot Spots Across The Country A Comprehensive Map
Apr 29, 2025 -
Georgia Deputies Shot During Traffic Stop One Killed Another Injured
Apr 29, 2025
Latest Posts
-
Solve The Nyt Spelling Bee April 1 2025 Complete Guide
Apr 29, 2025 -
Nyt Spelling Bee Puzzle April 3 2025 Answers And Strategies
Apr 29, 2025 -
Nyt Spelling Bee April 3 2025 Complete Answers And Pangram Reveal
Apr 29, 2025 -
Nyt Strands Today April 1 2025 Clues And Solutions
Apr 29, 2025 -
Nyt Strands Hints And Answers March 3 2025
Apr 29, 2025